Cultivating AI for Good
Experts navigate a minefield of ethical challenges to apply artificial intelligence to society’s big issues
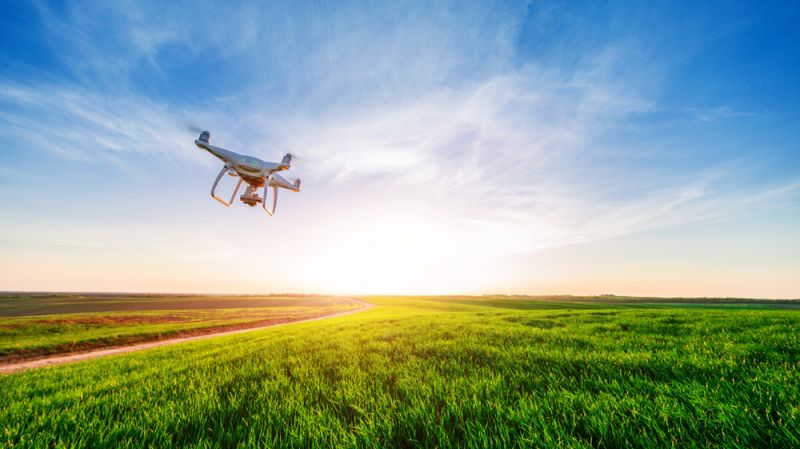
We live in a time of slaughterbots and other autonomous weapons controlled by artificial intelligence, of AI-driven deepfakes undermining political institutions. It’s easy to despair at how disruptive technology is wielded for such dark ends. But it’s worth remembering that technology does not (yet) have consciousness and can just as easily be used for humanitarian purposes.
If you need reassurance that all is not lost, there is a growing movement of white-hatted technologists bent on applying AI to society’s most frustrating challenges. AI for Social Good (AI4SG), for example, builds interdisciplinary partnerships centred on AI applications that align with the 17 Sustainable Development Goals of the United Nations. The lords of Big Tech—firms such as Google, IBM, Intel and Microsoft—have initiatives that target social needs. Educational institutions such as Smith School of Business do their part by laying the ethical and technical groundwork for a future generation of AI practitioners.
The suite of technologies under the AI banner—particularly natural language processing, speech and audio processing, and computer vision—has an extraordinarily high ceiling for innovation in the social realm. Early applications include helping people with atypical speech to be better understood, fighting illegal poaching with purpose-built AI cameras, and using image recognition and classification to detect plant diseases and soil deficiencies.
“With AI, there are biases and ethical issues that come up, but it also has huge potential in areas like health care, poverty alleviation and homelessness,” says Tina Dacin, the Stephen J.R. Smith Chair of Strategy and Organizational Behaviour at Smith School of Business. “People in the AI for Good movement are saying, How can we deploy this technology in a way that actually makes a meaningful social impact on society?”
For its part, Smith’s AI “ecosystem” of graduate programs, research, specialized labs and practitioner partnerships is nurturing the socially conscious side of AI. In partnership with the Scotiabank Centre for Customer Analytics (SCCA) at Smith, Dacin launched the AI for Good initiative at the school.
Dacin also teaches a course in the Master of Management in Analytics (MMA) and Master of Management in Artificial Intelligence (MMAI) programs that explores the intersection between analytics/AI, ethics and society. Each year, students work in teams to develop a viable prototype based on using AI for social impact. The SCCA kicked in funds that are awarded to teams judged to have the best projects.
Some past projects have applied natural language processing, image processing and voice recognition to identify racial bias in hiring interviews; AI and analytics to optimize the Canadian immigration application process; and conversational chatbots to help people affected by intimate partner violence.
On the research side, Smith scholars such as Ceren Kolsarici, Ian R. Friendly Fellow of Marketing and director of the SCCA, and fellow Smith researchers such as Nicole Robitaille and Laurence Ashworth, are working closely with government agencies and companies on AI-driven projects with a focus on sustainability and societal impact. These include increasing adoption of online tax filing, decreasing energy consumption across businesses and increasing the uptake of financial advice, particularly by financially vulnerable parts of the population.
Kolsarici has also been involved in leveraging AI-driven insights to help with Kingston’s local organizations, notably the Kingston Tennis Club, which has benefitted from several initiatives to improve operations and club success.
AI tools are accessible
Part of the excitement in the AI for Good community stems from the accessibility of the technology. Plenty of the tools used to train AI models are available on cloud-based platforms, and they’re getting less expensive and easier to use every year. Open-source AI libraries have simplified many of the tasks that used to require backend knowledge of AI algorithms.
AI for Good applications are also getting a boost from companies that have invested in in-house AI infrastructure and developing algorithms for profit. “They can use the same know-how that’s already generated to help vulnerable populations or segments that are not as rich in resources,” says Kolsarici. Doing so, she says, “these companies can generate good for society and also create a positive brand association.”
While the availability of cloud computing and open-source AI libraries will undoubtedly make it easier to design AI applications for social good, there are still significant barriers that bedevil all AI-based projects. Data accessibility is often the biggest one: essential data may be privately owned or controlled by a government agency that may not be willing to share the wealth. Social enterprises also compete for AI technical talent against tech companies and other for-profit firms that can promise higher salaries.
Ethical risks are no less significant. Dilemmas involving data privacy and bias embedded in AI models are magnified in social and humanitarian contexts. And the continuing challenge of making the outputs of machine learning algorithms transparent and explainable hampers their acceptance and use.
What is good enough?
Even with mitigation, there will always be tension in deciding whether an AI for Good application is appropriate to develop. For-profit innovation is guided by the adage, “Don’t let perfect be the enemy of the good.” For AI applications designed for a social purpose, “good” may not be good enough.
“There’s always going to be a downside to any type of technology and implementation,” says Dacin. “There’s also going to be a transitional period in which we’re not sure the efficacy or the impact that can occur. But here’s the dilemma: Let's say it’s right 83 per cent of the time and it’s wrong 17 per cent of the time. What’s the threshold at which we’re comfortable to proceed?”
It’s a question that comes up when Smith faculty assess the AI for Good student projects. One that triggered a lively discussion involved the use of children’s drawings combined with facial recognition technology to analyze the children’s emotional state. According to the proposed project, the results would guide potential interventions to treat depression or other mental health issues. The proposal generated mixed reactions: one judge thought the idea was worth developing further rather than cutting off innovation prematurely; another said the project made them uncomfortable and could risk stigmatizing children with mental health issues.
Smith students are taught to address such tensions during the design phase of their projects with a heavy dose of empathy. This requires asking questions designed to put themselves in other people’s shoes. Who is being served or harmed by using the AI solution?
“This type of work,” says Dacin, “relies a lot on deliberation and intentionality.”