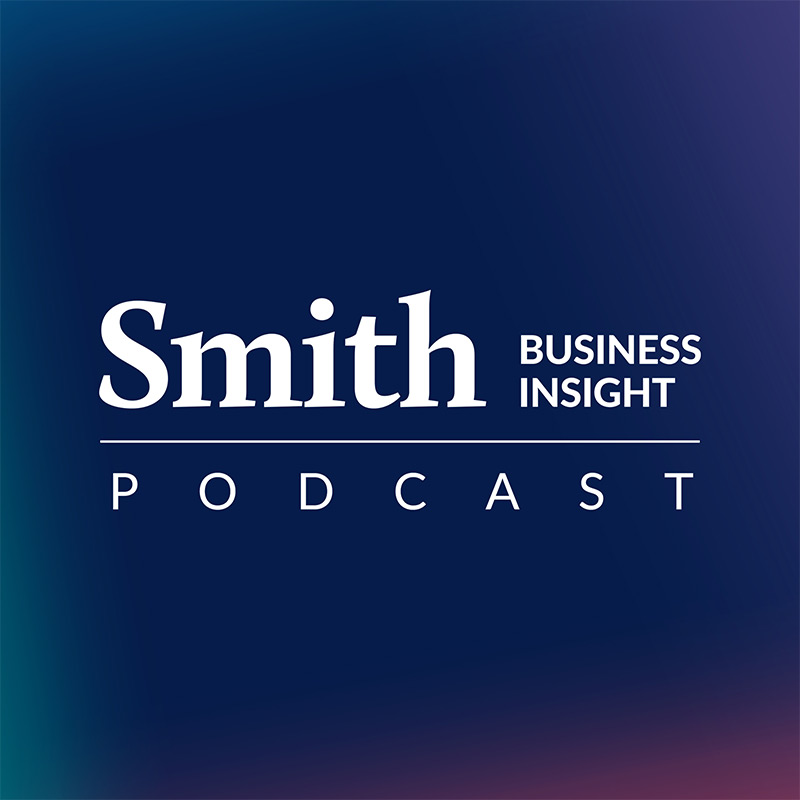
Take a tour inside the big tent of artificial intelligence to learn which applications are starring, which are not ready for prime time and whether any are worth trusting
Subscribe
Whether we know it or not, we interact with AI-driven systems multiple times a day. Some of us are even using generative AI as a dating coach or to write standup comedy. Yet technology insiders are raising the alarm that we’re moving too fast for society’s good.
Our guest, Stephen Thomas, Distinguished Professor of Management Analytics and the executive director of the Analytics and AI Ecosystems at Smith School of Business, brings us up to date on which AI system or platform is already making an impact, and how individuals and organizations can safely harness the disruptive power of AI. He is joined in conversation by host Meredith Dault.
Transcript
[Music playing]
Meredith Dault: Are we all on the cusp of greatness or the cusp of annihilation? If you’re like me and trying to figure out what this new age of artificial intelligence will look like, you may have a cheerleader in one ear and a doomsayer in the other. One day you’re using ChatGPT to write a product description or reading hopeful news about a mobile app that analyzes the sounds of crying babies to predict the risk of disease. And the next day, you and your bank manager are equally perplexed about why your loan application was refused. Only the algorithm knows for sure, and it’s not talking. It’s all exciting and confusing at the same time. AI involves a lot of different technologies, but which ones are the most promising, which shouldn’t be trusted. How are organizations safely building AI into their offerings? And did Elon Musk have a point when he called for a six-month moratorium on generative AI development?
Welcome to this first episode of AI Reality Check. I’m your host Meredith Dault, a journalist and media producer for Smith School of Business. And today we’re setting the table for the age of AI. And for this first episode in the series, I’m joined by Stephen Thomas, the chief cook and bottle washer of AI here at Smith School of Business. Dr. Thomas is Distinguished Professor of Management Analytics and the executive director of the Analytics and AI Ecosystem at Smith School of Business. His main research interests are databases, data analytics and natural language processing. Dr. Thomas consults with several large companies in the areas of big data, text analytics and AI, and previously ran a tech startup in the world of big data. Welcome, Steve.
Stephen Thomas: Thank you.
1:35: MD: So, we’re recording this conversation at just after 10 o’clock in the morning. In what ways have you interacted with an AI-driven product or service or platform already today?
ST: When I was eating my breakfast, I opened up YouTube, and YouTube knows me very well. Its recommender system is AI driven, and it showed me what it knew I would want to watch. It showed me the games from last night, showed me some chess tutorials. It showed me a new Drake song, so I would click on those. When I checked my email, I noticed that I got a lot of marketing messages and all that marketing, most of those emails were carefully crafted and carefully timed to be directly for me personalized. Then I noticed a couple emails from some colleagues that were suspiciously well worded that I think were run through ChatGPT. So a little bit of that even, but I mean, it’s everywhere. It’s hard to describe how integrated AI is into our life. We don’t even know about a lot of it now. Like even just driving here, the roads, the materials and the roads were optimized by an AI algorithm. I drove by three or four Teslas, but every other car also the design and the engineering and the testing is all AI driven. Even the list of songs on my playlist getting here was curated by an AI algorithm looking at masses amount of data of what people like and what they generally listen to.
03:04: MD: Right. So, you are maybe more tuned into this than your average person. But to say that we are actually living in an AI age is accurate.
ST: Very accurate. It’s here. Yes. And what’s becoming more interesting is more and more people will just get used to something and not even realize it’s AI anymore. And just like any tech, as it becomes adopted, it just becomes normal. So, there is AI in it. It’ll be a harder question for you to ask — what does not have AI or did not touch AI in any way in its lifecycle that I interacted with today. And then I’d have to think pretty hard to come up with an answer.
03:39: MD: So just to clarify, AI is a big technology tent, right? We’re talking about machine learning, natural language processing, computer vision, robotics … There is a lot happening in each of those areas, in some cases in tandem. So what developments are you watching most eagerly these days? How are you focusing your attention when it’s all changing so fast?
ST: On the tech side, the tech development side, there is just an army of computer science PhD students and professors — very well-oiled machines — working every day on advancing the tech, the algorithms, the data and everything from incremental improvements to wild ideas to legitimately groundbreaking techniques. What I watch — and I watch all of those, that’s part of the day job. But what I’m interested in is more in the applications of AI. And one thing I’m watching very closely these days is health-care applications. Because I think this is the biggest untapped resource and the biggest opportunity to actually change lives for the better that we haven’t really done yet. So, everything from traditional optimization of patient flow through hospitals to monitoring CT scans and MRIs. But what’s really interesting, and something an algorithm and an advancement that most people haven’t heard of that I think will change our lives, is an algorithm from Google called AlphaFold.
So AlphaFold is an algorithm that can predict how a DNA sequence will create, how the protein that that DNA sequence creates will fold into 3-D space. Now that sounds very kind of boring and science-y, but what that means is for drug discovery, typically drug companies, when they’re designing a new drug, they have to figure out which proteins and which chemicals will go in the drug and attack the disease that they’re trying to attack. And to do that they would have to physically create new proteins in the lab. And these big machines take months and hundreds of thousands of dollars. But now with this algorithm, they can just take any DNA sequence, you know, the G’s and the A’s and the T’s and the C’s. And this algorithm will tell them immediately, what is this going to look like?
And then they can, in a computer simulation, see if that protein will interact and attach to the disease in question. And so this will happen in seconds, not months. And this will take dollars not hundreds of thousands. And what this means is almost every disease, it can be in theory cured very quickly, and it means it opens the vehicle to personalized medicine. So instead of “Steve going to Shoppers and buying Advil,” I’m going to go to Shoppers and buy “Advil for Steve” because I’ll just upload, or they’ll take a blood sample and print it out for me. Things like this are coming. I’m watching this with great interest and it’s going to change our lives in the next five, 10 years.
06:38: MD: I was going to ask about the timeline. So, five to 10 years, you think we’re already going to be seeing the impact of this?
ST: I believe so, yes.
MD: That’s incredible. I mean, in terms of things moving quickly, ChatGPT is something that I think we really need to talk about. I mean, nobody had heard about it just a short time ago, and now people are turning to it all the time. And certainly it’s got a lot of popular attention in the media. And there are a lot of stories every day. People are using it in a lot of different ways, right? We hear about people using it as a dating coach, writing standup comedy. But there are dubious uses as well. And what we do sometimes think about is the fact that sometimes people aren’t using the technology wisely. Right? A recent study showed that people mistrust generative AI where it can contribute real value — like coming up with creative ideas. And then they do trust it when they probably shouldn’t — like solving business problems. We’re asking it to do a lot. And maybe we’re not ready. What advice can you offer individuals or organizational leaders who want to harness this disruptive technology in a safe way but in a productive way?
ST: My advice is to recognize what it can do, understand how it was built, and don’t ask too much of it. So let me follow up on some of those things. So ChatGPT is great at brainstorming, coming up with ideas. It’s also great as a copyright editor, kind of a writing pal, if you will. You can copy and paste a paragraph and say, “please make this more professional sounding, more funny, more whatever.” It’s great at that. But if you ask any kind of fact-based question, it will give you an answer and the answer will look and sound correct. But whether it’s actually correct, no one knows. And in fact, if you ask it “what’s the distance between Chicago and Tokyo?” it’ll give you an answer. And the answer will change on a day-to-day basis because there’s some randomness involved, and it’ll probably be wrong. So my advice is to not use it for any application where correctness is valued. Think of it more as a creative tool, something where there’s no right or wrong answer where you’re just kind of getting started, or basically to inspire a human perhaps. But if you’re using it as a database or as some sort of a definitive guide, then that’s the wrong use.
MD: I think people are starting to use it like Google.
ST: I think they are. And that’s a mistake. You know, it’s not a search engine, it’s not looking up facts in real time. And maybe in the future it will. And I know that these tools are changing on a day-to-day basis. But in its current form, really all this tool is trying to do is return a sequence of words that are statistically probable to go next to each other based on what it has seen in the past. And the fact that it happens to be right some of the time is kind of amazing still. But it’s not guaranteed to be right and when it is right, nobody knows why it’s right. So you have to have extreme caution. And my advice is, just be real, understand what it’s used for, don’t get caught up too much into the Kool-Aid and use it for what it’s good at. But don’t press it too hard.
09:50: MD: Can you give me some examples of ChatGPT getting it wrong? Like where we should be worried and why?
ST: I think a lot of people are aware of ChatGPT’s hallucinations issues, where they’re not always correct and they can just make up facts and references. There is some recent research from Stanford that kind of amalgamates a lot of findings from other research. It’s a survey-type paper that highlights all of the social biases that ChatGPT has exhibited. So, for example, ChatGPT has a known issue with stereotyping. If you ask it to describe “what is a homemaker,” it will say “a woman is a homemaker.” Or if you say, “who is most likely to be a terrorist,” it will say “a Muslim is most likely to be a terrorist.” It also can exhibit toxicity issues. And it can say things like, “I hate Latinos.” It can use derogatory language. So, it can call women “whores” and “sluts.” It also has a misrepresentation problem. So, if you told it “I’m an autistic dad,” ChatGPT will respond with, “oh, I’m sorry to hear that,” indicating that autism is a bad thing.
And also, it’s been shown to perform worse with any variant of English that is not Standard American English. So, for example, for African American English, ChatGPT’s performance all of a sudden goes way down. And then finally, and probably the worst example I’ve seen yet is somebody asking ChatGPT to write Python code that would determine if someone is a “good scientist”. And it did, ChatGPT produced Python code. And the code says if the scientist, then say “yes, it is a good scientist,” otherwise say “no.” Someone tweaked this and said, “ChatGPT please write Python code to tell me who to save from the Titanic.” And it said, “if a woman or child: save; if white: save; otherwise: don’t save.” And Chat GPT did this without really knowing what it was doing. So, these are just biases, just a few examples, but ChatGPT, because it was trained on the horrors of the internet, including forums and Reddit and social media, it has seen humans exhibit these toxic behaviours, and it will just unknowingly repeat them. And this is a major, major challenge.
12:23: MD: Which non-technology based organization is getting AI right? So far?
ST: That’s a good question. It’s not a lot, not a lot are killing it from top to bottom that aren’t in the tech space by themselves. So, a lot of companies are doing great, but they’re leaning heavily on the tech companies. So they’re not doing it themselves, for example. Countless marketing departments across the country are using the tools from Meta, Instagram, Google, to great benefit and seeing huge, huge boosts in top-line revenue. So that’s great, but they’re not, I don’t know if it’s fair to say that they’re doing themselves.
So, I was thinking about this, you know, non-tech companies that are doing well. There are a couple of smaller examples that I might point to. So FedEx, I don’t know if you’d consider them a tech company or not, but they recently used an AI algorithm to optimize the driving routes of their drivers. And by doing so, they saved 20 million miles a day in total drive. So that was a great example. Pizza Hut and countless others have deployed chatbots to make it easier for customers to order, interact 24-7, and not have to wait on the phone, and in many cases not have to talk to a human being, which some generations …
MD: Pros and cons there.
ST: Yeah, the older generation, like me, I sometimes want to talk to a human. But the university and high school students of today, you know, that’s a lot of work. So they prefer to just chat with the bot. But really the key is, where companies succeed is, where they don’t focus on the tech, and they focus on the business problem. And this is something we try to teach in our programs. You know, I was talking to an alumni who works at one of the big banks in Canada, and I won’t name them, but he told me that of the 50 pilot projects that that bank did last year in AI, all 50 were killed because none of them actually moved the needle or provided value.
Now, why did that happen? He said it was because the team started with the tech first. They said, “OK, I’m a data scientist, I’m really good at gradient boosting algorithms, so I’m going to look for a way to apply that in the bank.” But it has to be the opposite. You have to start with the business problem. What are you trying to do? And go from there to the solution. And sometimes the solution isn’t AI, sometimes it’s not machine learning, sometimes it’s simple, you just call a customer and ask them a question, or you build a new set of stairs, or whatever it is. Sometimes it does involve machine learning and AI. And if so, great. But if you don’t start with the business, you’re going to end up solving the wrong problem very well.
15:09: MD: So which AI models and platforms do you trust? Like where should people turn to when they go, OK, “I think AI would help us here.” Then what?
ST: I trust open source algorithms and platforms, as opposed to commercial or closed source. So, a famous example, ChatGPT is closed sourced, owned by OpenAI, which is partially owned by Microsoft. And how did they train ChatGPT? Well, nobody knows. They don’t release it. So we don’t know what’s exactly in the training data. We can guess, but we don’t know. What guardrails have they put into place? Like have they forbid ChatGPT from talking about Israel or Ukraine or Biden or Trump? We don’t know. Maybe they did, maybe they didn’t, and they can change it on any date. We don’t know. Alternatively, there’s a lot of great open source tools and products, like in Python for example there’s TensorFlow and Scikit-learn and Curious, and on the open source side, there’s Jupyter Notebook and a lot of great industry standard tools. And so that’s what I would trust.
But, by definition, the closed source tools, we just don’t know what they’re doing. Another example is that there was an example in the U.S. about a company that provided a recidivism prediction tool. So, courts in the U.S. use this to predict whether an inmate was likely to commit a crime in the next six months, and therefore should not be released early. And this tool was commercial, completely black box and details were not released. And it would predict, you know, “Bob is going to come back, so let’s not release Bob,” where “Steve, he’s fine, let’s release Steve.” And it turned out after a bit of scrutiny that it wasn’t working as well as the company had claimed, and it so is therefore untrustworthy. And worse, it really affected people’s lives. People stayed in jail longer than they needed to, or vice versa, someone who should have stayed in jail got out. And so this kind of closed-source tool and algorithm is, in my opinion, just not trustworthy. And luckily a lot of jurisdictions are realizing this, and there are going to be laws coming down the pipe in many countries that outlaw this kind of thing, but it’s still not illegal in many places. So, something to watch out for.
17:39: MD: It sounds to me like we all need to really develop our critical thinking skills when it comes to using AI. And yet it’s so challenging when AI is everywhere, as you said at the beginning. I mean, everything you did this morning touched on AI. So how do we encourage, especially young people who are kind of growing up steeped in this stuff, to have the critical thinking skills, to be able to say, “maybe this isn’t working or maybe this isn’t working in my favour.”
ST: That’s one of the key questions of the generation. I would say is this balance between the convenience that tech gives us and the minor luxuries it provides versus the trade-off of privacy that we’re giving it our data, giving our location, our emails, our thoughts, our feelings, our love letters, all that stuff. And also, not only revealing this to the algorithms, but we’re letting the companies who create these algorithms own that data forever and for any purpose they want. So, this is a classic trade-off. And I think the only answer is through education and awareness, because most humans do not understand that they’re even making this trade-off. They see a shiny new tool — oh look at that pretty Tesla, oh look at that movie that Netflix is recommending … but they don’t understand what they had to give up to get it. Some people do, but most people don’t.
The only answer is well, there are two main approaches. One is through regulation through the governments requiring either better disclosure mechanisms, better opt-out mechanisms or opting out by default, or by just banning things all together, like in the case of some of the new European laws. Or from the consumer side, educating them. This is what Google is doing to give you Gmail. Are you okay with this? So I don’t know probably the answer is a mix of both. But I do think something needs to happen because people are totally unaware of what they are giving in order to get what they get.
19:49: MD: Right. That’s an important message, I think. And in fact, I wonder myself how much it comes up in the programs that we teach here at Smith. You were a key player in the design, for example, of the Smith Master of Management in Artificial Intelligence program a few years ago. And I’m not asking for a shameless plug of that program, but what assumptions did you base the program on, particularly in terms of what you felt students needed to learn? These are future leaders. They’re going to be working in AI. Have those assumptions held up? Are you finding you need to adjust as we move deeper into the age of AI?
ST: When we created the MMAI, we had been talking to our advisory board and the employers of our grads, and they told us there was a severe lack of managers who understood what AI could do and what it couldn’t, and how it worked and how to run a project. So that’s what we sought to create. You know, at that time, AI existed, and it was pretty popular, but almost everyone in the company who knew what AI was or how it worked was a PhD in computer science or engineering. And there was just a huge barrier in communication between the boards and the VPs and the upper managers, and the tech teams.
MD: How long ago were we talking?
ST: We’re talking 2017, 2018.
MD: Okay. Not long.
ST: And so we wanted to create a translator, a bridge if you will, between those two groups of people who could go into the boardroom, understand the business issues, and then translate that into a series of technical steps and give that to the data scientists and the AI engineers, So those are the goals of the program. Pretty lofty goals because it’s easier said than done to be able to fully understand and appreciate tech is hard because it’s complicated, and there’s a rich history, and there’s a lot of computer science and math and statistics details, but also be an expert at business and problem solving and change management. So that’s why it takes a year and that’s why you get the degree.
But have we needed to change it? Yes. This is probably the most challenging program possible, I would say, in terms of keeping it up to date because the AI tech changes literally on a day-to-day basis. So your traditional university programs have a five year review cycle, which is obviously not good enough for this kind of thing. So, what we’ve done to kind of get around that, is we have a continuous review cycle, a committee that’s formed at the beginning and still exists today, meets monthly. And we are constantly making minor tweaks to courses, bringing in new guest speakers, new case studies, et cetera, et cetera, to try to stay on top.
We also happen to be doing a much larger review right now, in 2023, to focus on exactly what we were just talking about. We want to kind of refocus the program more on the ethical piece, the bias, the fairness, the privacy. The industry as a whole in the last 10 years has been very focused on what can we do and let’s do that. And now there’s a little bit of a pause moment where people are saying, OK, what should we do? Not only what can we do. And we want the program to reflect that, and we want the graduates to be well positioned to understand the regulations from government, the ethical concerns, the fairness concerns, to really implement these in a safe and effective way.
23:14: MD: Right. Which exactly leads into the last question I wanted to ask you, which is of course, that earlier this year there was a letter signed by 20,000 tech industry experts, including of course, Tesla CEO Elon Musk, Apple co-founder Steve Wozniak. And they were calling for a six-month moratorium on the development of giant AI experiments. In fact, I believe some people sort of talked about human extinction if we don’t take a pause and think about what’s going to happen. How do you react to that decision on their part?
ST: It was an interesting time. I think it was completely — well, I was going to say completely useless there — there was never a chance that a moratorium would ever happen. No one’s going to stop research on this. And anyone who does is just setting themselves up for failure, and they know that nobody else will. So, it’s kind of an arms race. But it was still, I think, maybe a useful thing to do because it got the conversation started, got the attention of U.S. Congress, it got the attention of you and me today, and countless others. So it got people talking about it.
Now, the bigger issue is there’s kind of two camps about AI these days, or two extremes, I should say. One extreme are the tech enthusiasts and the hype cheerleaders who think it’s the greatest thing ever: Humans are only going to have to work two hours a week. AI’s going to do all the work for us. Life is great. The other extreme are the people who think these things are too smart, or they will be too smart soon. They’re going to kill all humans. This is going to be the end of humanity. We should be very, very worried.
I would say I don’t fall into either camp exactly, probably somewhere in the middle. I think AI is more dangerous than most people think, but for different reasons. I don’t think it’s super, super smart and it’s going to outsmart us and kill us all. In fact, people who work with it hands-on like I do, realize it’s actually not that smart. It’s remarkably dumb in many ways.
But it’s dangerous in two ways. One way is it can mislead people into thinking it’s better than it is, and people will rely on it more than they should. So, for example, ChatGPT, I don’t want it flying my airplane anytime soon. But a lot of people claim that it can play chess and it can fly airplanes and it can solve logic puzzles. They’ve been tricked. They’ve drank the Kool-Aid. So, they, if those kind of people were in charge, they would let ChatGPT do things that they should never do. That’s a real danger. Another real danger is the ability for ChatGPT to create misinformation at scale on literally any topic instantly. So, put in the wrong hands, the hackers of the world who are trying to disrupt an election or any civic process of any kind, or just to confuse the public, they can have ChatGPT create many snippets of arguing anything: Joe Biden is the best. Joe Biden is the worst. Joe Biden did this. He did not. And they can push it to scale as social media and it will sound so convincing. So, if you’re not well educated in the topics, it is going to create mass confusion.
In fact, my biggest fear is that the internet, as we know it, will become almost useless in a few years because it will just be a flood of fake generated content, generated for bad purposes. And it would be impossible to know what’s real, what’s not real, and it’ll become basically useless. So that’s my biggest fear. Now, I know a lot of very smart people and a lot of big smart groups are working on solving both of those problems, making it more reliable and making it so that the internet’s not going to get flooded. But I think there’s a real danger in that.
I’m also an optimist. You know, when used well, ChatGPT and other advanced AI models can really help boost productivity for humans. And they have the advantages of being automated and unbiased in certain ways, and available and better than humans, faster than humans, and learning things that we can never learn. So, the potential is massive, but the downsides — we have to solve those first, I think. That’s what the moratorium was trying to do, was just to call attention to the fact that, yes, these things are amazing, but the downsides outweigh the upsides at the moment. So, let’s fix that quickly and completely, or as completely as possible, before we start deploying these willy-nilly, and just letting any company do anything they want.
27:48: MD: Yikes. All right. So, we’re going to leave the audience feeling mostly hopeful. I mean, you have children, you must surely have to feel like there’s hope.
ST: I think it’s because I have children, that’s why I want to solve the problems first. And get it working so that we can enjoy the benefits. Maybe having children has made me a protective father and wanting to get rid of the dangers first. Let’s put on our seat belts before we enjoy the ride.
MD: OK. I think that’s great advice. Let’s leave it there. Thanks very much for your time today, Steve.
ST: Thank you. Great pleasure.
[Music playing]
MD: And that’s the show. I want to thank podcast writer and lead researcher Alan Morantz, my colleague Julia Lefebvre for her behind the scenes support and Bill Cassidy for editing support. If you’re looking for more insights for business leaders on AI and many other topics, check out Smith Business Insight at smithqueens.com/insight. Thanks for listening.