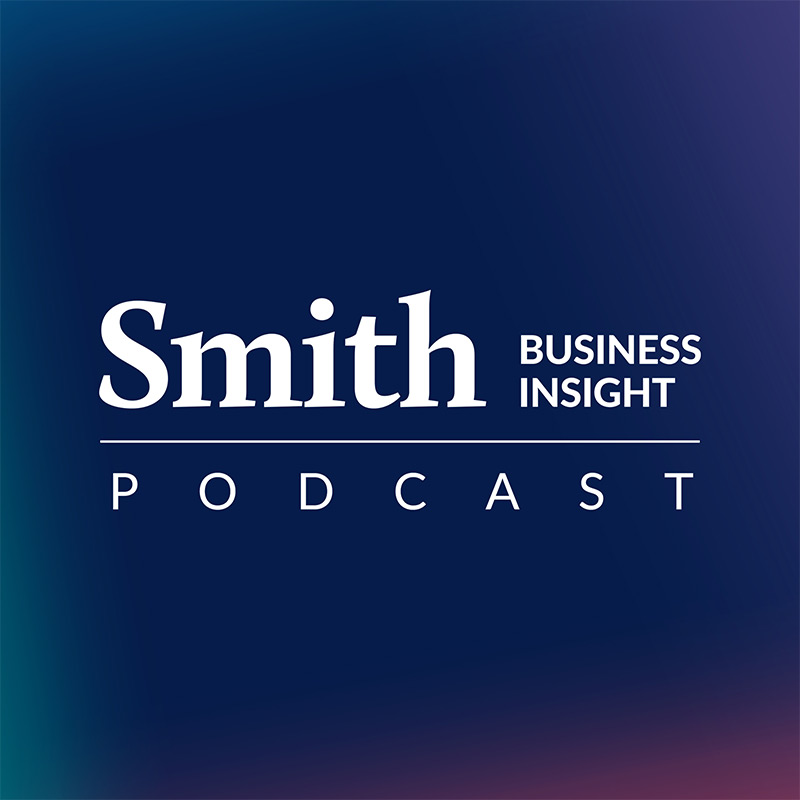
Algorithms hobbled by human biases are playing havoc with people’s lives. How do we best respond to the challenge?
Subscribe
From high school students given the wrong marks just as they’re applying to university to Black defendants misclassified as higher risk for reoffending, AI is driving unfair and damaging outcomes. Technology firms promise what they call Responsible AI. But can they really deliver if they can’t keep up with the speed of change? Can governments impose ethical standards and safe use of AI-based systems and products?
In this episode, Anton Ovchinnikov, Distinguished Professor of Management Analytics at Smith School of Business, discusses his groundbreaking research into the government response to algorithmic bias and what happens when large language models are fed AI-generated synthetic content rather than human-generated content. He is joined in conversation by host Meredith Dault.
Transcript
Meredith Dault: Imagine graduating high school students, being assigned the wrong marks, just as they’re applying for university. Or how about women being blocked from consideration for technical jobs at Amazon? Or black defendants being misclassified as being at higher risk to re-offend? Well, these have all happened thanks to the handiwork of rogue algorithms hampered by biases, though they were clearly not ready for prime time, that didn’t stop them from being ruled out to play havoc with people’s lives. The age of AI promises transformational change, but not all of it positive. Large language models, which you know from ChatGPT, are being trained using human created data that’s biased and the problem will only get worse in coming years. Technology firms promise what they call responsible AI, but can they really deliver if they can’t keep up with the speed of change? Can governments impose ethical standards and safe use of AI products? Should they?
Welcome to this episode of AI Reality Check. I’m your host Meredith Dault, a journalist and media producer at Smith School of Business, and today we’re talking about ethical AI. My guest is Anton Ovchinnikov, Distinguished Professor of Management Analytics at Smith School of Business. Dr. Ovchinnikov’s trailblazing research has been published in leading journals, and his papers and case studies have won numerous academic awards. Before starting his academic career, he worked in Germany, the Netherlands and Russia in the area of commercializing high-tech developments. He also co-owned a business in industrial and architectural design. Welcome Anton.
Anton Ovchinnikov: Hello.
01:40: MD: Anton, let’s go back to the early days of Covid, so back to the spring of 2020. It was a big mess for all of us, as you can remember. And particularly for the millions of high school seniors who were unable to do their end-of-year exams at school. As a result, their schools had to figure out other ways of determining their all-important final grades. Grades that would, in a lot of cases, chart their academic futures. As I alluded to in the introduction, the International Baccalaureate Organization decided to use an algorithm to set those final grades. I know you’ve studied this case. Can you pick up the story from here and let us know how it worked out?
AO: All right, so indeed. Now imagine the situation, right? So, the International Baccalaureate Organization, IBO, it’s a large international high school program that operates all around the world. And as a result, they not only had problems at each individual place, right? But they also had a problem across the countries, right? So if one country would allow to run exams, another wouldn’t allow to run exams, right? Then how would the grades be compared? So what they decided to do is they decided to create a model that would take all the data that they have about a student — and they had a lot of data — they have run, I believe, for almost 50 years in something like 150 countries. So there are a lot of students that went through their programs, right?
So they thought of taking that data to create a model that would predict the outcome of an exam, given the students’ performance up until the schools were closed for Covid, right? So it turned out that, I mean, several things to note here, interesting things to note here, is that the organization by itself seemed to lack the capability to do so. So they outsourced it to an unknown third party who actually did the modeling work. And what we know is that that model worked very well on average, that it was very well calibrated to produce results consistent with prior years. However, for very many students, it produced kind of borderline nonsensical results. And the case study that I wrote is in fact exactly about that, right? That this model that seemed to be doing well on average, really was not doing good for almost anybody, right?
So some students received grades that were higher than everything that they had received in the past. Other students received grades that were lower than anything that they had received in the past, right? And of course, if you realize that this is a super major decision that changes a person’s life, right? What you will do, where you will live, oftentimes who you will marry, right? These type of things are determined by where you go to college, right? And so, this is kind of an example of an enormously high, high stakes decision that was delegated to the model. And in this kind of stressful situation, it didn’t work very well, right? So, there were borderline protests. I believe that there were petitions to abandon this that were signed by something like a quarter of the student population at the time.
U.K.’s prime minister Boris Johnson called this a mutant algorithm or something like this, right? So kind of again a big, big scandal. Now, in terms of how it eventually worked out, eventually they kind of created a process to fix the most ridiculous outcomes. Let me put it this way, right? That’s really what happened. Now this is a big story, and again, because it was so early in a way of an AI development, you can think of this as probably the first global AI crisis, right? That affected many people in a serious way all around the world. But there were many things that were lacking. For instance, there was no very good way to appeal machine decisions. There were good ways to appeal teachers’ decisions or grades, right? But there was no way to appeal something that was done by an algorithm. Which I think would be an important thing for us to go forward is how exactly do you appeal a decision that was made by the algorithm and not by human.
05:45: MD: And then ultimately the outcome wasn’t fair for the students. And that raises this question that fairness is often mentioned in relation to AI. But what does that mean for you? Where does fairness come into this when it comes to machine learning?
AO: So it’s a broad and somewhat loaded question, but let me first answer a slightly different question, or make you realize a slightly different question, is that with this world of algorithmic decisions, we can actually ask questions about fairness in ways we could not have asked them about humans. So, in some sense, this algorithmic fairness — we are asking these fairness questions at a higher level than we would have asked for humans. Now, why are we doing so? We’re doing rightfully so because an algorithmic decision is very easy to scale. So now think of this IBO example that we just discussed, right? Once the model was created with one click of a button, there are 70,000 students who were affected, right? If you would have had, let’s say, a manager or a bureaucrat somewhere, applying some kind of questionable fairness that maybe they would have done 10 cases or something like this. But, but it’s not very easy to scale. It doesn’t scale monumentally, right? These AI systems, they scale right away, right? Very, very quickly. And so that’s why we are treating them to somewhat higher standard, but also because they’re replicable. We can repeat the same thing. We can say, how would it work this way? How would it work that way? We can in fact do these investigations of algorithmic fairness in ways we could not have done it with people.
07:21: MD: So can you give us some other examples of where fairness and AI are colliding?
AO: Of course. So, in fact, I have done some work about lending practices. In particular, gender bias in financial services. So, for example, if an algorithm knows that you are a woman, is it fair that, let’s say, you are given extra credit or you are denied credit? That’s one of the questions that my work investigated.
MD: So, in other words, a complicated issue.
AO: It is a complicated issue. But what I want to point out is that I’m actually very glad that we are exploring this as a community, I think as a society even. And I’m also very glad that we all realize that with algorithms, we can ask these questions more rigorously, we can study them more than we could do with people.
08:12: MD: Well, let’s talk more broadly about the explosion of ChatGPT into our worlds. As you know, over the past year, we’re seeing more of it and more people are relying on it. And you were part of a research team that looked at whether ChatGPT makes the same sort of bias decisions that humans make. Can you talk about what you found?
OD: Yes, with pleasure. So in fact, let me preempt this question a little bit by just understanding how big of a scale we’re talking about here. So we did a kind of literature review to see what people are saying. And Gartner, a technology consulting firm, they predicted by 2060, 100 million people will have AI colleagues on their teams, OK? Think of what that means.
MD: That year again, 2026
AO: By 2026, right? So just two and a half years, yeah, two years and a bit, right? A hundred million people will have AI colleagues. Artificially intelligent workers, co-workers on their teams. And so now of course, what does it mean for the biases, right? So we studied biases in humans for maybe 70 years or so since, since the 1950s. But now we have these non-human agents on teams with other humans, right? So that’s why we want to study these biases right now. This particular example shows how the biases of these models could propagate kind of within an organization, but then the value chain partners could be using these models.
Walmart, for example, designed an AI tool that negotiates contracts with its vendors and, very interestedly, vendors actually prefer to negotiate with an AI tool than with the Walmart employee.
MD: Why? No emotions?
AO: My sense is that the answer is a little bit different. The answer is that you don’t really need to chase it. It kind of does things fast, right? That’s my intuition for why people prefer it. That, you know, you send an email and they don’t respond for five days, right? No. The algorithm kind of, you submit what you want and it responds to you right away.
Let me give you another point to emphasize why studying these biases is so important. So what percentage of North American adults you think consulted with ChatGPT about financial advice?
MD: Oh, boy. I don’t know. I hope it’s not high.
AO: So the last I saw was 54 per cent. So think about it. Half of, wow, half of North American adults asked ChatGPT for financial advice.
MD: And…
AO: Well, what’s the risk? But that’s exactly why we need to study all the biases, right? So, for example, in biases of humans, we talk a lot about risk aversion or loss aversion, right? So basically how people rightfully so should consider the variance, let’s say, in financial returns in addition to the expected value of financial returns, right? I would say that a person with a very large portfolio could kind of survive a big variance, especially if they have a very long horizon, right? But people with smaller portfolios, so maybe with smaller time horizons, they really should care about variance in addition to the expected return. So guess what happens with ChatGPT? ChatGPT applies this variance and expected returns very much in a hierarchical fashion. It’ll first look for opportunities that maximize expected results, and only if they’re equal, it’ll then consider variance. So, in other words, it’ll kind of allow people to quote-unquote, buy an almost unlimited number of variability in their returns for a very small increase in expected return. And that, of course, it’s very different from how people would consider risks, right? And it is somewhat dangerous, right? Especially for, again, people with smaller portfolios or maybe with a shorter timescale in their investments.
12:12: MD: So we’ve been talking about generative AI and associated with this technology are these large language models that are trained on massively large data sets. And up to now, the raw material for these programs, as you’ve alluded to, has been all digital content that we humans have created over the years, which is a lot, a lot of content. Surely there’s a time when even that’s not enough to fuel more advanced AI products. Presumably these large language models are eventually going to be fed AI generated synthetic content rather than human generated content. And what does that mean for the integrity and the accuracy of future AI products?
AO: That’s a great question, and I wish I knew the answer. So I will again respond to two slightly different questions that I nevertheless hope will help. So, in fact, maybe even three questions. So first, we are indeed reaching a situation where there will be a lot of AI generated content. And the problem is that currently there is no very good way to detect it, right? That is specifically for language, right? The text that is written by humans and the text that is written by these advanced LLM models, they are undetectable. There are no good ways to say which of them was written by a human. Right? Now, this is a problem of active research and people are working on it, but so far I have not seen results that have found a convincing enough degree of discrimination between the two. They cannot classify which one’s which.
Now, with images and sound, things are a little bit easier because there is a way to embed certain patterns in the images of sound that would not be detectable by human eye or human ear, but would, nevertheless, it’s like a signature that is embedded in an image that you cannot see, right? But an algorithm can see, right? So that’s what happens there now, but specifically to your question, so will these models be trained on this AI generated content? I want to kind of flip it in a very different way. These models are called large language models, and the kind of naive connotation is that the language is text, but the real revolution behind these models is to rethink the meaning of language. So these large language models are not trained just on text.
The earlier ones were trained just on text. The current ones are what’s called multimodal. So they’re trained also on images. They’re also trained on videos. They’re trained on DNA data. They’re trained on stock market data. They’re trained on weather patterns, MRI data and, and so on and so forth. In other words, I personally don’t think there’s a real shortage of naturally generated data, that is not AI generated data, that could be used to help train these models. So, in some sense, your question is a little bit more of if there is quote-unquote a pollution of the data with an AI generated data, that this data may get into training models. But at the same time, there are many sources of data that we now understand are like language that are nevertheless not written or spoken language in, in a colloquial sense.
So in fact, let me give you an example, because again, the audience may not realize this, but be interested in this. So now you probably have seen these generative AI models that generate images like DALL-E, or Midjourney or Stable Diffusion, something like these types of models, right? So think of how they work. You put in a prompt in text, let’s say in English, and it gives you an image, right? So I want everybody to realize that this is a form of translation. You put a text in English, it gives you a text in French, that’s translation between English and French. You put a text in English and it gives you a picture. That’s also a translation between the meaning of this text in English and the meaning of the same text in an image.
16:12: MD: So, “an apple on a table”, it has to interpret what I mean, and it gives me the translation in a visual.
AO: Exactly. An apple on a table in French–la pomme sur la table–or whatever right? But in an image, it would be an image of a table and an image of an apple, right? But that’s translation. It’s translation from one kind of medium, which is text, to another medium that is image, right? But images are still language. It’s like a visual language as opposed to spoken or written language. So these large language models. I think it’s important to understand that the meaning of language extends far beyond the written language or spoken language, right? So all of these different forms of data are language and all of these different kinds of translations between them are forms of translations. And this is why, in fact, this is why these models have seen such a big improvement in performance recently, because the scientific community was able to rethink the meaning of language and the meaning of translations.
17:16: MD: Then where does the bias come into play for this stuff? Because we know that bias is inherent in these learnings.
AO: Oh, yeah. So it’s a good, good point. There are two sources. So let’s go now to a simple form of language like the written and spoken language, right? So again if people are biased, what they wrote or how they wrote it, is biased. So that’s one. It just comes from the training of the model. But then all these models have a next step to it. So once the model has been trained, there is like a step two. That step is called reinforcement learning with human feedback or from human feedback. And in fact, that’s like a secret source for the ChatGPT, for example, a massive improvement in performance. So, how does it work? Once the model was trained, Open AI hired a whole bunch of people that interacted with their system.
So they asked the question, they got in the base case, they got four responses, and then people said, which of those responses they liked the most, right? So think: you’ll get four responses, A, B, C, D. And then that person who was involved in interacting with ChatGPT said, “OK, I like D the best”. Then let’s say, I like A, which is about the same as B, and then C is the worst, right? So this kind of input, that is human feedback, right? This human feedback was then embedded in the next layer of training the model where the model tried to kind of try to have its already pre-trained language model in such a way that it produces more of Ds, right? And less of Cs in my particular example. And that is a place where bias can also come in, that is with real people who were looking at the texts that were generated by a machine and saying, “oh, I like this one more than I like that one,” right? In fact, some of the research that we have done suggested that it is actually that part that created more bias — maybe not more, but some of the bias is almost one hundred percent due to that second part. But let me emphasize it. That second part is sort of the reason why these models are so good. Because they were trained to produce outputs that are kind of quote-unquote liked by humans.
19:31: MD: Right? And then it makes mistakes. I mean, I guess the question I’m trying to get at is what happens when it’s taken that information? It’s now got some biases, and then we’ve got a world that’s sort of building information based on these biases that are inherent in the system. Do we end up in a just a big soup of misinformation?
AO: Well, a soup of misinformation is a slightly separate topic. And let’s talk about something first, and then we’ll come back to the misinformation. OK?
Now think of the role of educational institutions, right? So historically there was a value of knowing something. I think with generative AI tools, the value of getting an answer to something, it’s easy to get an answer. You know, you type a question to ChatGPT, it’ll give you an answer, right? So therefore the value that our students can add, that our graduates can add in the society is being able to critically assess that answer and say, well, is this a good answer? Is this right? Now, of course, here, I realize that these models are trained to produce text that is deceivingly good, right? So therefore, this is not an easy task. So, this is not an easy task that they’re kind of by design, very articulate, right? And that puts more emphasis on humans, who are interpreting these outputs to say if this is in fact a correct answer, right? Have there been some features that were not considered, some important features of the situation that were not considered?
Now, let’s go back quickly to the misinformation, since you asked. So an analogy that I want you to think about is, think about counterfeit money. Canadian money is now plastic, so there’s a piece of plastic, right? So why does this piece of plastic have any value? Because we as a society have trust that if I have this piece of plastic, then I can go and exchange this absolutely useless piece of plastic to something that is actually useful for me, for food, for transportation, for any whatever. Right? Now, imagine if we now would have a lot of counterfeit money that everybody would be able to print their own dollars that look deceivingly, like the actual dollars, right?
Then the trust in the system would very quickly disappear, right? And then people would stop, basically, there would be no need for me to carry the $20 bill, right? If there’s essentially no trust that I can exchange this $20 bill for something useful, right? So we need to do the same for AI and misinformation, right? So in very serious ways for how the governments are really prosecuting let’s say printing of counterfeit money, right? We should think about the same, for example, about prosecuting creating artificial people, right? On social networks, for example. So we should think about how do we make sure that this particular $20 bill is a real bill? We need to ask the same question. How do we make sure that this particular person who is engaging in a discussion is in fact a real person, right?
22:36: MD: But that’s not easy.
AO: Well, that’s not easy, but realize that three years ago we did not have that problem at all, right? And the counterfeit money is a problem since, I don’t know even what were the earliest examples, right? People were shaving off a little bit from the diameter of a silver coins and then minting new silver coins, right? From what they could shave off, right? OK. So anyway, it took us several hundred years to fix, more or less, that problem, right? And it’ll take us some time to fix this problem, hopefully sooner. We’re now a lot more empowered to do that.
23:08: MD: Yeah. So on that question of government, we know that governments have been trying to figure out how to respond to AI through policy and regulation. And, in fact, Canada just launched a voluntary code of conduct for firms using generative AI as a stopgap between now and when the proposed artificial intelligence and data acts come into effect. You and Stephanie Kelley, who was a Smith PhD student at the time, looked at what happened when governments tried to address the problem of fintech lenders refusing loans to women because of those biased algorithms, and it backfired big time. Can you walk us through those findings?
AO: Yes, with pleasure. So let me step back a little bit. So the title of the study which kind of summarizes in many ways, what we do is “Antidiscrimination Laws, Artificial Intelligence, and Gender Bias.” All right? So let’s kind of unpack this title. So in almost every developed country, there are anti-discrimination laws that date back to times when people were discriminating against other people, right? So in the United States, for example, in financial services specifically, this law is from 1979, right? That’s certainly not the time when AI decisions were kind of on a big scale, right? So in a way, there are these laws that were designed to make sure, to make it harder, I guess, for people to discriminate against other people. Now 40 years later? These laws are applied to decisions that are now done primarily by algorithms and not by people, right?
So therefore, these laws, they kind of mess up a little bit with the model training, with the data governance and several other steps, right, in creating of an AI system. And so what we do in this research paper is we look at the typology of these laws around the world, again, specifically applied to lending decisions, right? And then we say, so what do these laws actually do in the present day situation when these lending decisions are done by algorithms? Alright? And specifically, because we want to look around the world, we were kind of deliberating about what kind of feature would we select, right? And like, let’s say North America, a racial issue probably would be a big one, but realize that racial issues are very different around the world, right? So therefore, we looked at gender, right? Something that is relatively universally understood kind of across the world, right? Okay.
So now going back to these anti-discrimination laws. There are, roughly speaking, three kinds. So Singapore is one of the most liberal countries in that sense. So all the data that country has can go into the training of the algorithm and can be used in the ultimate decisions. But the organization owns the outcome that this organization can be called responsible for, let’s say, using or not using gender data in their lending models. On the opposite end of the spectrum is the United States, where for the non-mortgage decisions, organizations are prohibited not just from using the gender data in their AI models, but even from collecting it. That is, let’s say a credit card company in the United States would not know which of their applicants are women and which of their applicants are men.
Europe and Canada are somewhere in between, where the organizations can collect that data, but they cannot use that data in a specific individual’s lending outcomes. All right? And we show, somewhat paradoxically, the laws like in the United States, the most restrictive laws, they actually hurt women, right? And again, now think about it for a minute. So it is a situation where a company does not know if you are a woman or a man, right? But not knowing whether you are a woman, or a man ends up hurting women rather than helping them. And of course, back in 1979, the connotation was that, well, if a person is reviewing your application and they don’t know who you are, you don’t know your gender, right? Then they will not discriminate against you. In fact, many times when we presented this, we got this, kind of like, people were staring at us to say, how can you discriminate against women if you don’t know who women are?
27:24: MD: Yeah. I think I still need you to unpack this, I don’t really understand. So in not knowing they’re women, they’re discriminated against more. Why?
AO: Why? OK, let me give you three facts. Let me say three things, which are all true. And we’ll start building the story from there. So first, all women are better borrowers than men. They’re more creditworthy. Fact number two, all people with more work experience are more credit worthy than people with less work experience. Fact number three, women on average have less work experience.
So basically, now think of what happens. If an algorithm does not know who is a man and who is a woman, and then let’s say there are people with three years of work experience, right? It would either give all of them loans or not give all of them loans, because it cannot differentiate between women and men, right? But imagine a situation where the algorithm would now know that, let’s say, this is a woman with three years of work experience, and this is a man with three years of work experience, the algorithm can then give a loan to a woman with three years of experience, but not give the loan to a man with three years of experience, right? And so as a result, by depriving the model from knowing this, the model will learn about creditworthiness from other things. And in the world where the majority of borrowers are men, all these kinds of, let’s say, work experience of a woman would … the model will learn from a work experience of a woman much more as if that would be a man, right? And so that’s what ends up hurting women in our particular situation.
29:08: MD: These kinds of algorithms are increasingly being used to help make decisions around the world, right? So should we be worried?
AO: So, let me give you one more example and then answer a question. So, a famous example in this case is Amazon, right? They used algorithms to read resumés and then recommend who to interview and who to hire. Very similarly to our setting, right? The majority of software engineers at Amazon are men, right? So therefore, the things that were good for a man, they were, let’s say, perceived as being strong indicators of strong performance, right? So now imagine if a candidate who went to a women-only college. Of the star software engineers at Amazon, there are not many people like that, right? So therefore, going to that college was kind of thought of being a problem. That certainly is not a signal of a very strong performance, right? Because in your dataset, there are not many data points like this. Or being involved in, or being a captain of a women’s soccer team. Again, you will then say, OK, how about a women’s chess club, right? So how many of my top software engineers were in a women’s chess club? Oh, not really very many. So that’s probably not good, right? From that perspective.
But of course, the point is that being a captain of men’s soccer team, or men’s chess club —probably more related — would be an important feature, right? And so again, kind of depriving from knowing who were men and who were women ended up hurting women, kind of in a very similar case. Now, going back to your question about whether we should be concerned well, the short answer is, if we do nothing, I think we should be concerned.
But kind of the silver lining in our conversation is that it’s not that we’re doing something, right? We’re doing something both in scrutinizing these algorithms more than we scrutinize humans just because they’re easier to scrutinize. I mean, after all, they’re the technical algorithms. We kind of know almost exactly how they work and realize that we have almost no idea how the brain of a human really works, right? And so we know a lot more about these systems than all this legislation that is coming into play now. The fact that a lot of this data is open and people from all over the world are training these models and looking at it in different things. So, in other words, if we do nothing, I think we should be scared, but we’re not sitting doing nothing. We’re in fact doing a lot.
31:38: MD: So as ChatGPT grows in popularity, and we see it getting rolled out and used more, by more and more individuals, and more and more businesses, how are you feeling as we look at the future?
AO: I like it.
MD: You like it. You're feeling optimistic?
AO: I like it. I think so. So well, what was one of the projections? That something like 30 per cent of work will be done by these tools in the next however many years. Now, of course, here, we need to be concerned about the entire sections of work being done by these tools, but realize, that in a lot of people’s lives, there is really 30 per cent of useless work that they’re doing during the day. So if that work can be now done by a machine, that’s perfect. OK? And so I am actually quite optimistic. I think that these tools will allow us to become more productive. It’ll allow us to free more time.
Now, how will we use that time? Of course, that’s a question that we need to think about, but again, in an interesting way that kind of changes which professions are important, what should we be doing. So if we free up this time to spend more time with our children, maybe, you know, we grow more, or with our aging parents, right? Who are expected to live longer and longer, right? Maybe that’s not necessarily a bad thing. If more people, you know, run around, breathe fresh air, go to the gym, become healthier and happier, then I think it’s a fantastic kind of use of technology.
MD: I’m going to take that optimism and run with it. Thanks, Anton. It’s been a pleasure speaking with you today.
AO: Absolutely. Thanks so much, Meredith.
MD: And that’s the show. I want to thank podcast writer and lead researcher Alan Morantz, my colleague Julia Lefebvre for her behind the scenes support, and Bill Cassidy for editing support. If you’re looking for more insights for business leaders on AI and many other topics, check out Smith Business Insight at smithqueens.com/insight. Thanks for listening.