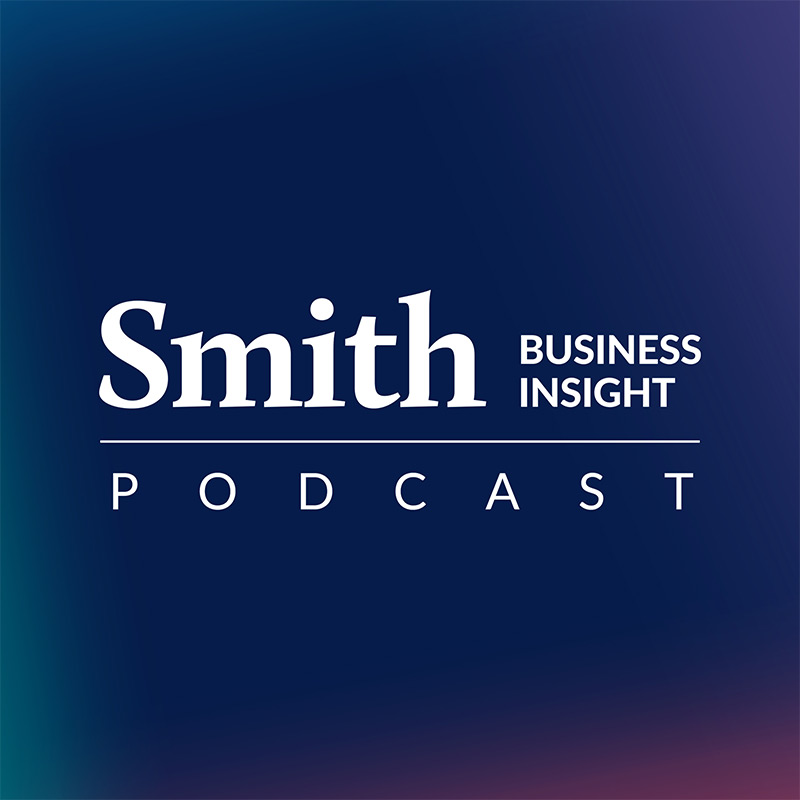
Say hello to the newest member of your team: Cathy Cobot. And goodbye to predictable team dynamics
Subscribe
While AI will surely take over some jobs lock, stock and barrel, the more likely scenario is that we will have to share our jobs with AI entities. It can be challenging enough to work with fellow humans and understand their emotions and thinking styles. What will it be like working with super-smart non-humans? Will it change how we behave or make us question our judgment? Will it keep tabs on our performance?
This episode explores these questions with guests Tracy Jenkin, an associate professor at Smith and a faculty affiliate at the Vector Institute for Artificial Intelligence, and Anton Ovchinnikov, Distinguished Professor of Management Analytics at Smith. Dr. Jenkin and Dr. Ovchinnikov discuss what they’ve learned so far from their research that explores human-AI collaboration and cognitive processes. They are joined in conversation by host Meredith Dault.
Transcript
[Music playing]
Meredith Dault: I know more than a few people who are feeling apprehensive about some AI entity taking over their jobs or about a future where they have to work with robo colleagues. Maybe it’s a well-founded fear. After all, how will we share tasks? And will doing that actually make me question my own judgment? Will the tech be keeping tabs on my performance for my boss? And can I ask the robo colleagues what they did on the weekend?
Well, there are lots of researchers out there trying to get answers to many of these very questions. That’s because while AI will surely take over some jobs, lock, stock and barrel, and I say that as someone who writes for a living, the more likely scenario is that we humans will have to share our jobs with AI. It can be challenging enough to work with fellow humans and understand their emotions and thinking styles. What will it be like working with super-smart non-humans?
Welcome to this episode of AI Reality Check. I’m your host, Meredith Dault, a journalist and media producer at Smith School of Business, and today we’re talking about human-AI collaboration. We have two guests today from Smith School of Business who know a thing or two about AI in the workplace: Tracy Jenkin and Anton Ovchinnikov. Dr. Jenkin is an associate professor at Smith and a faculty affiliate at the Vector Institute for Artificial Intelligence, and Dr. Ovchinnikov, who was our guest in Episode 2 of this series, is a Distinguished Professor of Management Analytics. The two are currently working on a couple of research projects that explore human-AI collaboration and cognitive processes. Welcome Tracy and Anton. It’s great to have you here.
Anton Ovchinnikov: My pleasure.
Tracy Jenkin: Great to be here.
1:43: MD: So I’m going to put this to both of you and then I’ll just let you take turns responding. Humans have been collaborating with technology since before the Industrial Revolution. What makes this AI collaboration different?
TJ: Well, if we think about it, we’ve been working with systems over the past, say, 20 or 30 years. Much of that has been sort of automating things that we already know how to do. So we know how accounting systems work and we can do the addition, et cetera. However, it’s just a lot of work. So we get them to automate that to make things go faster and smoother with artificial intelligence. And the tasks that we’re asking it to do, in many cases, we are asking it to potentially work with much more data. We’re asking it to do things that are much more complex. So things that we might not be able to do ourselves; to generate some sort of insights and patterns across multiple different dimensions and variables that the human brain can’t process. So we might not know what the right answer is, and that’s the difficulty, to be able to look at the results and assess: “Is that right?” And that’s particularly difficult if it’s different from what we expect.
MD: Got it. OK. Anton, do you want to weigh in?
AO: I think that the AI systems are just a lot more capable, and that’s what makes things different. Now they’re also capable in a way that is different for us. I think that if you think about this historically or evolutionary, so going back from the hunter-gatherer societies, we had bulls to help us on the field and so on. So these were like powerful devices that were making us stronger. And so we evolved, right? This happened over hundreds of years. Then we had machines, ships, automobiles and whatnot, airplanes and all the kinds of industrial machinery, if you can think about that. But we really never had brain machinery — something that extends us intellectually. And that’s what’s different.
3:46: MD: Right. That’s a good way to put it. So can you give us a big-picture view of the different schools of thought and the big questions that are being asked by researchers who are studying human AI collaboration?
TJ: I’ll maybe start with the fact that one major branch of research is looking at how humans adhere to the recommendations of these AI systems. And what we’re finding is that, in some cases, there is aversion or algorithmic aversion where individuals just don’t want to adhere to the recommendations of those algorithms, those AI systems. They’d rather listen to and adhere to the advice of humans or themselves even though the algorithms outperform humans.
On the other hand, there’s also algorithmic appreciation where individuals will just go along with the AI or algorithm’s advice even though it might be wrong. And so that’s one large branch of research. We’re looking at explanations. That’s another branch to research. So in many cases, it’s a look at how can we make algorithms interpretable and have some sort of explanation. It’s a black box, the algorithm, so we don’t know how it made its decision.
In some cases, the nature of the algorithm itself can be interpreted. For example, a decision tree. We can follow along down the tree branches to understand what factors influenced the outcome, the decision. In other algorithms, like a neural network, it’s very black box. We don’t know how the inputs got translated into the outputs, meaning the decision or the recommendation. And therefore we want some sort of explanation to say, “Hey, how did you actually come to this decision?” so I know why I was granted credit or I was highlighted as a fraudulent case. So that’s another branch. And beyond that, when do we want to have those explanations for those recommendations? And so that’s part of the research that we’ve been looking at — when do individuals seek explanations from algorithms differently from human versus AI advisers?
AO: On my end, let me put another kind of branch of work on the table, which would be delegation. Think of this as an analogy to a tractor. A human decides that they want to go work in a field, and they go to the field and start a tractor, essentially saying, “Hey, let’s go and do some work.” Now, with AI tools, it’s very unclear whether it should be a human in the driver’s seat and then asking AI for help when the human wants it, or if AI should be in the driver’s seat and asking humans for help when it wants it.
And, in fact, there is some work, not our work but some other work by colleagues at a different university, that showed that it’s the second approach that usually works better. That is, AI asking humans for help rather than humans asking AI for help. And very interestingly in their study is this notion about how well do you know what you know. And it turns out that machines can be very well calibrated. That is, when they don’t know something, they would realize they don’t know it. While for humans, it’s very hard to be calibrated. Humans, essentially, are not very good at knowing when they don’t know something and therefore asking for help in those situations.
7:09: MD: Can we sort of get into the weeds a little more around what AI-human collaboration actually looks like or could look like? Any use cases you want to point to so we really understand the big picture?
AO: Yeah, of course. Let me go first. Now think of planning or purchasing something. Numerous organizations need to decide how much of certain stuff to buy or book. That could be procurement of inventory in retail, that could be booking the number of nurses that you need to have on a particular day and so on. So these kinds of tasks, they, generally speaking, fall under the decision-making of uncertainty, right? Had I known for sure that I would need 17 nurses between 5:00 and 7:00 next Wednesday, I would book exactly 17 nurses. But the problem, of course, is I don’t know exactly how many nurses I need. And that’s the problem. So now it’s a forecasting task, right? And in these forecasting tasks, a collaboration could take the form of the following kind: an AI tool gives a projection about how many nurses it thinks would be necessary. And then the human can either adhere to that recommendation immediately — that is, the algorithm says you need 19 nurses, and then the human says, sure, let’s just go and call 19 nurses to work on Wednesday. Or a human can change that recommendation to something else. So that’s one possible scenario.
MD: OK. Do you want to weigh in Tracy?
TJ: Yeah, and it’s an interesting question because there are so many different flavours of AI systems out there. So we can probably spend a long time thinking about this, but I’ll add on to Anton’s example where we’re trying to predict a specific number. There are also algorithms that would specify a category or decide whether an event is likely to happen or not. So we can even think about that in the medical context of whether a patient has a particular disease; or in the business context, whether we think a particular case is fraudulent or whether we think a customer is likely to churn, meaning they’re going to move from our organization to another organization.
The other thing that we’re seeing now with the advent of generative AI, so things like ChatGPT, which probably many of our listeners would be familiar with and probably have tried out, where in that case, with generative AI, we can interface with it in natural language, and that either produces an image or produces some text. It might answer a question that we have in natural language or produce content in natural language. So, for example, I was recently needing to think of a title for a presentation. So I asked ChatGPT, just to help me get the creative juices going. I also sometimes ask it to help me with code if I’m unfamiliar with how to call a specific package or just need an assist on how to frame a particular piece of code. And so those are just additional examples of how we might be collaborating with these different forms and new forms of AI.
AO: Let me add two more. One is kind of generic and one is from a recent personal experience. So think of these generative AI tools as a way of lowering the cost of intelligence. That is, now it is cheaper to do a task with these tools than with a human doing the same task. Now, what does it mean? It means that we can actually scale intelligence. Going back to my previous example, imagine if we are not just staffing nurses, but let’s say you have a whole bunch of different positions, and let’s say nurses are an important position. Therefore, a manager may be thinking about this a lot. But let’s say there is another position that is a lot less important, and so a manager would naturally not really think about it much, or maybe think about it, let’s say, only once a year.
And there would be the same five people of a particular kind who are always invited to work in a particular shift. Again, with these tools, we can scale intelligence. We can apply the same data-driven rigorous thinking to staffing nurses that we have done in the past to staffing everybody in our organization. And a very similar example could be extended if you think of inventory items. You have some big items that sell a lot, and usually they attract a lot of attention. But there would be a lot of other items that sell very little. And oftentimes there is actually very little thought going into how to deal with them. Again, with these AI tools, we can scale intelligence. The key point here is that the cost of intelligence has decreased, therefore we should apply it more.
Now, if you want yet another example, here’s a personal one. So I have a rental house, and one of my tenants was moving out, and they asked for a letter of reference to show to their landlord in the next place where they’re moving to. And I asked ChatGPT to help me with that reference letter. And before that, I had thought for a few minutes about what I would write in my letter. And non-surprisingly, many of the things that I was thinking about, ChatGPT also suggested. But it also suggested something that I had not thought about on my own, in particular, the fact that the tenant was very good at communication. And I thought, wow, that’s a very good point. I, as a landlord, would love to know that the tenant notifies me when there’s a problem right away and that the tenant responds quickly if I want to send a technician to repair something. So these kinds of things, again, you can think of them as augmenting intelligence, right? But that’s essentially like a second opinion on something. Eventually, I wrote a letter. To be honest, I found ChatGPT’s letter to be a bit too exaggerated; not the way I would have done it. But the key point from this example is that there was this one thing that I did not think about, just again because I gave it like five minutes of thought. So both of these examples fall to this extending intelligence.
13:14: MD: And, of course, when it comes to writing, nobody likes a blank page. So why not get the computer to do that first draft for you?
AO: Well, to be honest, in that case, that was not particularly helpful. That is, what it wrote, I essentially had to delete it. But the point is that it allowed me to expand my intelligence. And again, intelligence became cheaper. Maybe I could have consulted with another human about writing this letter. I hope you see my point. As the cost of intelligence is going down, we need to have more of it. That’s kind of basic economics 101.
MD: Yeah. That’s great examples. Thank you. I’m hoping the two of you can now just for our audience delve into a little bit of the work that you have been doing on the subject of human-AI collaborations. I understand you have a couple of projects on the go. Tracy, do you want to kick us off by telling us about some of these projects?
TJ: So we’ve got two research programs that we’ve been working on. The first one is more directly about AI and human collaboration. And that’s where we’re trying to understand when do humans seek explanations, and do they do it differently when they’re asking a human adviser versus an AI adviser? And then, how do they adhere to that recommendation? Do they show some aversion? Do they show appreciation? What are the differences there? And so in that case, what we found is when an individual is presented with an unfavourable and an abnormal case, they will more likely seek an explanation from a human than an AI adviser.
What we also found is that for the adherence to the recommendation, they sort of go the opposite way. When it’s a favourable recommendation, and they ask for an explanation, they’re much more likely to actually adhere to the recommendation of a human adviser. On the other hand, for an AI adviser, when it’s an unfavourable recommendation … So think of this as you have thought about a particular price for a rental unit of an online property rental system, and the adviser recommends that you lower your price. So you’re like, I don’t really want to lower my price. That’s unfavourable. And when you get that advice from an AI adviser, you are more likely to adhere to that AI advice than a human adviser.
15:38: MD: So you think that the AI adviser’s more trustworthy in that instance?
TJ: That’s what we found. And we found some other interesting things from looking at the comments from the participants in that they weren’t really asking for explanations from these AI advisers very often. And in those cases, we deduce that the reason was because they trusted the AI adviser more. But some interesting outcomes were that they felt that in some cases they might not get an explanation from the AI adviser. Can AI actually give you an explanation? And if it can give you an explanation, am I really going to understand what that explanation is? Am I going to get a big mathematical equation that I’m not going to really understand or that’s not really going to make sense to me?
MD: So rather than questioning it, just go with it.
TJ: Exactly. Go along with the recommendation.
MD: OK. Did that surprise you? Did those results surprise you?
TJ: Yeah, I think they did … I see Anton wanting to jump in here too.
AO: I think that in some sense they did, but in some sense they didn’t, right? So the mental model that we have in this research and for what’s going on in a person’s head is a three-step process. So step number one: Am I really so surprised that I want to have an explanation? Then step two: If I ask for an explanation, will I get an explanation? And then, if I get an explanation, will I be able to understand it? And what we find is that there are distinctions in all these three steps between humans and AI. And perhaps the last one is maybe the least surprising in the sense that you are more or less confident that with an explanation from a human, you will be able to understand it. With AI, of course, I think more people doubt if they will be able to understand it.
The more surprising is the first step, and that’s what Tracy was alluding to. What people find surprising when they get it [advice] from a machine is different from when they get it from a human. And here is perhaps this — so Tracy will help me with the exact name for it — but here’s this belief of machine superiority, right? Oh, it cranked all these gazillion data points and therefore it knows what it’s talking about. A human is still perceived to be somewhat of a high-level recommendation, an intuition-based recommendation that is maybe a little bit similar to mine, while the machine is sort of allowed to be very different because of this superior analytical ability.
TJ: Yeah. And let me just jump in there. There’s a concept called perfect automation schema. So that’s where we think the algorithm’s going to be perfect, right? It’s going to give us the right answer all the time. And so we might just trust in the algorithm because of that particular belief. The other side of that is when we see the algorithm err, we’re much more critical of it. So we think, OK, well, you are not supposed to err. If you do err, then we’re not going to trust you as much. We think you’ll continue to err in the future. Whereas with humans, we sort of expect that we’re going to make mistakes from time to time and we’re a bit more forgiving of that.
And just to build on what we’ve been talking about so far, what we’ve observed in the literature and what is built into our research model, an experiment, is that to know when an individual is going to seek an explanation, we’ve seen that it’s when there’s a surprising condition — there’s something unexpected and, in particular, it’s unfavourable. So that’s what we’ve been trying to study and when that differs in the human versus the AI adviser.
And so again, based on that notion of the perfect automation schema, as Anton says, it’s sort of in a way expected that if we see the advice or the recommendation from the algorithm is different from our expectations, and even if that is unfavourable, we kind of in our heads say, “Oh, well, that’s OK. It’s an algorithm. It must know what it’s doing. It must be the right answer.” And in our particular experiment, we were never giving the wrong answer. It was about the expected value of the outcome. So, even though it appeared that it was a lower price, the probability of success in renting this unit, for example, was higher. So the overall expected value of the outcome would be higher. And so, in these cases, individuals went along with that to say, “Oh, it must be right. It’s an algorithm.” But with humans, they weren’t as trusting.
AO: I think that the key point here is that lots of conversations about explainable AI and trustworthy AI assume that people would want an explanation, and that getting an explanation would be helpful for their ultimate decision. We are questioning that very, very core question. The very core notion is that sometimes people will not be interested in an explanation and, even worse, that sometimes getting an explanation can be worse than not getting an explanation. Therefore, at a very high level — and that’s where I think the implications are big — this research suggests that it is certainly not as clear that we should always provide an explanation.
20:54: MD: Ah, interesting. OK. While I’ve got you on the mic, Anton, do you want to start telling us about the second research program that you guys have worked on?
AO: Yeah, the second project that we also have with Tracy and one of my current PhD students, Yang Chen, is about the biases of ChatGPT. And so it’s another form of interaction between humans and AI. And we actually have sort of a mini research agenda there. So we initially started to look at just biases, and then we thought about how people will in practice interact with it. So we are changing that a little bit. What’s going on in that project is that we think of a typical business user in an operational context. Now, think about a mom and pop store, right? One that does not have a sophisticated automated system that the big firm would have and would therefore ask a generative intelligence tool like ChatGPT for advice about procurement or staffing or about some other operational decisions.
And so that is a form of interaction. In our current projects, we took again … In academic research, what you need to understand is that you are somewhat tied to where the literature has been up to now. So we took some of the formulations of these problems from the existing literature and we’re checking how ChatGPT would perform on them as opposed to humans. But in the next wave of this research, we’re thinking of even asking humans for these formulations because that again is another important question to study, right? So how will people ask the questions when they face a particular problem? You might have heard this notion of prompt engineering. And so how will people prompt the system to get the answers to the questions that they want? That’s the next wave of this research.
TJ: Yeah. And just to build on that, especially thinking about those listening to this session, if we think about some of the questions, if anyone has read the Daniel Kahneman book Thinking, Fast and Slow, those are some examples of the questions that we pose to ChatGPT. So there’s the conjunction fallacy or the sunk-cost fallacy, or framing those types of questions. And so we ask them of ChatGPT to see if ChatGPT demonstrates the same biases that we do as humans — and it might because it’s been trained on human data as well as tuned by humans. And so what we’ve been finding has been quite interesting in that in some cases it does show the same biases, in some cases it shows different biases, and in some cases it just is getting the answers wrong. What is also interesting is that as we’re sampling … So, for example, we might run the test 10 times or 100 times, meaning we’ll repeat the exact same question to ChatGPT to see if it consistently gives us the same answer. And it does not. And so that is very interesting too. So there’s a lot to unpack, I think, in this research, but it’s been very interesting so far.
24:12 MD: And what will you do with this project, with this initiative?
AO: As academics, the first and primary goal was to write academic research studies and submit them for publication to top, very high-level, leading academic journals. And in fact, our two initial projects are getting some good traction to very, very good, very highly regarded scholarly journals. Of course, after that, it’s what’s known as research mobilization or knowledge mobilization. It’s taking part in podcasts like yours to tell a generic audience about what this is. Again, so far, we started, as Tracy mentioned, just with these human biases that are known from the literature. So again, Thinking, Fast and Slow would be a great book that reviews many of them, right? And the reason we did that is because we need a comparative benchmark. For example, if we’re talking about, let’s say, framing bias or some cost bias, then we would like to know what humans have been doing. So that’s why we are, as I mentioned, somewhat tied to the studies that we’ve done previously with humans. We now are just redoing them with AI tools instead of humans.
MD: Yeah, it sounds like really exciting work. It seems so critically important right now.
AO: Absolutely. But as a next step though — and that’s where the continuation of this project is — what we really want to do is we want to give advice to managers; we want people to better understand how to interact with these systems.
MD: Right. So what does that sound like? Give it to us.
AO: The research is still ongoing. I think at a very high level there are certain biases that the systems do not have. That is, they are sort of behaving as rational humans should behave. There are certain biases where they behave much like humans we see kind of in the wild, quote-unquote, right? But most interesting, there are situations where they behave differently from both rational humans and actual human behaviour.
26:16: MD: Well, I mean, we laugh, but managers are standing by wondering, What the heck? What should we be doing? And what tools should we be using? And I mean, of course, we’re on Episode 6 of this series, and we’ve addressed some of these issues throughout, but certainly, we don’t have any firm answers yet. It’s still a work in progress.
TJ: Yeah. And I think the other part of this, what was interesting, is that in some cases where the tool got it wrong, where ChatGPT gave a wrong answer and it looked very convincingly right, but the math or the logic was actually wrong. And so that is a red flag in that, where do managers … how can they know when the answer is not correct? And when developers are developing these systems and taking the generative AI technologies and using them in their organization to be able to test for these, I think we’re going to see testing as a completely different thing from what we’ve known in the past where we’re going to have to be sampling and resampling and testing across different systems. It’s going to change, but I think that’s where we need to have some caution that it’s not always going to give us the right answer. But how do we know when it’s not?
27:27: MD: Right. And so if workers are fearful of AI, would collaborating with AI systems be seen as less fruitful than if they’re optimistic about learning new ways of working?
TJ: Well, just to add on to that, and this is again from my own personal experience. So how I’ve been using ChatGPT in the past couple of months is when I’m doing some programming. And so I may ask ChatGPT a question, and I can very readily check to see if it’s right, meaning I run the code. If it doesn’t work the way I think it should be working, I go back to ChatGPT and say, “Hey, that didn’t work. What went wrong?” And it actually realizes it made a mistake and it gives me another answer, or I go to other resources. But it might be that as we’re moving into these different applications, you know, to be risk aware of what we’re doing and have some safeguards so that we’re not making large wrong decisions. So I think to sort of have that hat on, that lens on and expand in a risk-averse or a risk-conscious way.
MD: Right. So as Anton says, augmenting my intelligence, not replacing it.
AO: I would actually say that, in my mind, there will be waves of how this would work. And so at the beginning, there probably will be kind of small things that are changing. But realize that over the longer time, I think the big things will change. And let me just kind of pause there and perhaps air a controversial point. Now, why do we need browsers? Why do we need an internet browser? And why do we need a website? Why do I need an Air Canada website? Because my brain cannot directly interact with Air Canada’s reservation system, right? And that’s why I need a computer browser to go there. Then I need their website to show me, to ask me where I want to go, what the price would be, what kind of seat I want to have, and so on, right?
But none of that is necessary if I can ask ChatGPT, “Hey, I want to go here. When are the best times for me to leave?” And so on and so forth. And it will just interact with the reservation system over here directly, completely bypassing the browser. That is, completely bypassing this kind of ad-serving capability that Google now lives from and all the other websites. Completely bypassing the website to begin with, right, Air Canada has no way of interacting with me directly and cannot show me a little banner of what the exciting destinations are. All the people who are working on designing that website and updating that website become potentially irrelevant and so on. So this is the kind of human and AI collaboration that can kind of cut complete slices of the world as we know it but ultimately will make things more efficient. Because again, if this type of tool is more like a personal adviser, it would know what meetings I have on that day and when do I need to be at my destination. So it’ll be able to kind of guide me or help me select a flight that is more relevant for me without me needing to think about it very much.
TJ: Yeah. And if I can just add on to that … If we think about this in our enterprise systems, the systems that we’re using in organizations and to run the organizations, could we also see a similar interface? Where we see a ChatGPT interface over top of our SAP systems, for example, our accounting systems, our logistics systems that will allow us to interact more in natural language to inquire about things or to actually get tasks done where we’re not typing things directly into the field. We’re having ChatGPT do that for us based on some input that we give it, and it might even be able to complete many of the transactions that we’re doing. So that’s another area that’s down the road somewhere, potentially.
31:23: MD: Right. So do both of you think that we have enough information to give advice to organizations on how to purposely design effective human-AI collaborations? Because right now we’re learning how to use the tools to our benefit as humans, right? But are we purposely designing effective human-AI collaborations? Are we ready?
AO: I don’t think anybody knows the answer to this question, to be honest. What I think is very clear is that being more knowledgeable about this, being more knowledgeable about opportunities, being more cognizant about risks, so essentially everything related to education and learning about this, is fundamentally important.
TJ: Yeah, and I’d echo that to say that becoming more aware and informed and learning about these systems — starting small, starting with small implementations, learning what are the risks, what can we leverage. I teach agile techniques in some of my courses, and so I think the agile sort of approach works, where we are experimenting, where we’re trying small experiments, where we sort of have a limited blast radius so that we’re not really being exposed to a lot of errors and damage, and just learning and having knowledgeable people. So one of the things I think about generative AI, it is very, very complex. And it’s easy to sort of see this superficial level of what we see it doing. But having a deeper understanding of what’s really going on underneath of that, learning about prompt engineering and some of these new techniques, and getting these skills into our organization … so as we are adopting, we’re just fully aware of what we’re adopting, how we can leverage it in our systems thinking and expanding our thinking too of what is actually possible. But becoming knowledgeable in the first place.
MD: So finally, what do you think it is that we should be thinking about broadly as we think about AI-human collaboration going forward?
TJ: So where Anton and I started our quest, our research on human-AI collaboration, we were really thinking about the fact that we didn’t feel that AI would be replacing individuals anytime soon, taking all our jobs. We felt that the important question was how can AI help knowledge workers. So how can individuals that are doing very complex tasks, how can they use AI to help them with a lot of the heavy lifting? We’re thinking about, you know, for example, a doctor. How can doctors leverage AI and increase what they can do when they have tools to help them in terms of the intellectual tasks that they’re taking? But with the advent of things like ChatGPT, we’re just seeing this massive general applicability across, I think, all facets of individuals, what they do on a day-to-day basis, as well as employees.
And I think that was unexpected. We didn’t really expect such a massive increase in how generative AI would allow natural language questions and writing and image creation to help us. And so, I think, it’s organizations, managers, individuals, just understanding these technologies and being creative, thinking out of the box of how they could do their work more productively but at the same time being aware of where it’s not going to help them. So when you’re asking a question, if you don’t know what the answer is, be cautious of what the answer is. Because with generative AI, it can sound very real. There’s a lot of risks out there too. So it’s not just a happy, everything is good and we need to just embrace it. I think we need to understand all of the pitfalls and the downsides and the biases and regulations, et cetera out there.
35:25: MD: OK. That’s a good reminder. And Anton.
AO: I think that first and foremost, we need to realize that the question of how humans should work with intelligent machines is perhaps the main managerial question of our time. We don’t have all the answers, we don’t even have all the sub-questions. But if we keep that in mind, that we spent hundreds of years learning how to work with other humans, we spent hundreds of years learning how to work across different cultures, the questions of ethics and related cultural issues … they all evolve. And our understanding all evolved in the context of humans working with other humans. Right now, we have systems that are reaching in their intelligence the intelligence of humans, and therefore we really need to rethink all of those managerial questions that we spent decades and decades and, sometimes with our evolution, hundreds and thousands of years learning about. We now need to redo all of that between humans and machines.
TJ: And just to add on to that, when we think about the jobs that each of us do many times, they’re very entrenched in those practices. And I think that’s sort of built on what Anton is mentioning that there are certain ways of doing things. And I think with AI, we need to sort of rethink how we do things. So just in the examples that Anton and I were both giving — Anton about how we are now booking with Air Canada, or how we’re writing a letter, or how we’re coding, or how we’re doing our work — we may need to rethink that and how our jobs look with an intelligent algorithm in the wings. How can we leverage that algorithm to help us do our job differently, but more effectively?
37:17: MD: I think that’s a great place to end. This concludes our series. I’m so really pleased that the two of you were able to lend your ideas and insights to this last episode. So thanks very much for being with me.
AO: Thank you so much.
TJ: Thanks so much, Meredith.
[Music playing]
MD: And that’s the show. I want to thank podcast writer and lead researcher Alan Morantz, my colleague Julia Lefebvre for her behind the scenes support, and Bill Cassidy for editing support. If you’re looking for more insights for business leaders on AI and many other topics, check out Smith Business insight and smithqueens.com/insight. Thanks for listening.