When Analytics Gets Personal
How to reap the benefits, and mitigate the risks, of prescriptive analytics
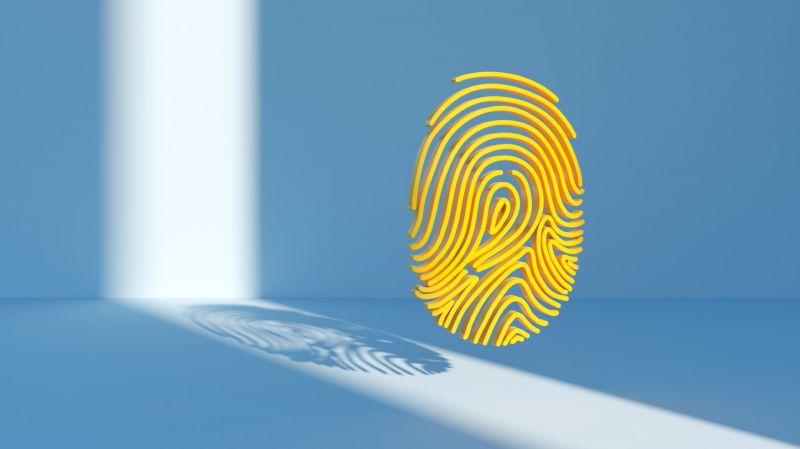
You hop onto Amazon to purchase a new vase for your home. As you scroll through the options, you come across the section “recommended for you”, which shows the styles you fancy, at great price points. The company knows what to recommend, and at what prices, based on your activity on the site as well as other customers’ shopping patterns.
This is prescriptive analytics at work.
At its root, prescriptive analytics helps companies know how to make decisions, such as pricing, more efficiently, and to navigate volatile markets.
“Amazon sells hundreds of thousands, if not millions, of products,” says Murray Lei, an assistant professor of management analytics at Smith School of Business. “There’s no human in the world that can correctly decide the price of these supplies.”
Prescriptive analytics has important applications across a variety of sectors — from helping companies develop and improve their products to helping banks identify fraudulent purchases. But this form of business analytics also raises challenging privacy issues.
“Prescriptive analytics is really a process that the company uses to make decisions based on data,” says Lei. “In general, I think of prescriptive analytics as being a larger part of data analytics as a whole.”
The analytics hierarchy
Lei says companies use different forms of business analytics to their advantage. Descriptive analytics, for example, helps firms understand their sales over the past month and the types of customers they have — essentially it covers existing trends. He refers to this as “step one” of data analytics.
Step two, Lei says, is predictive analytics, which involves using this information to understand future trends. “For example, companies can figure out if they charge a certain amount for a product, how many customers will they have in the next month.”
Lei describes prescriptive analytics as “step three”. It helps firms make decisions based on the data.
“Prescriptive analytics takes it to another level of understanding data,” he explains. “It takes this knowledge of customers’ behaviour and tells you what you should do and what decision you should make. Since you already understand customer behaviour, it tells you what you could do to maximize revenue, maximize market share and minimize cost.”
Privacy versus data breaches
Prescriptive analytics does have a significant downside: it carries privacy risks that affect customers’ personal information. One risk is a data breach, where consumer-level data is directly leaked. Lei says all forms of analytics are vulnerable since they all require data.
A second is that personalized decisions, such as recommendations and prices, can carry sensitive customer information. Lei says this type of privacy risk is unique to prescriptive analytics as companies may not communicate with customers about the output of other types of analytics.
“Amazon, for example, might predict you will buy a product at $10, with a 50 per cent chance of being right, but they most likely won’t tell you this. They just charge you a price based on their understanding of your purchase behaviour,” Lei says.
Algorithmic insurance pricing is another way that personalized decisions based on prescriptive analytics can be a minefield.
“An algorithm quotes different prices to customers with different health conditions, and it continues to refine its price recommendations as more and more customers come,” Lei says. “It may constantly tweak the prices for customers who have a certain disease but charge the same price for customers who don’t. An adversary [such as a hacker] can infer whether a customer has that disease or not by observing whether the price changes after that customer arrives.”
In other words, if the algorithm is sensitive to the behaviour of some customers in the database, then these customers’ sensitive information is at risk of a privacy breach.
To contain this privacy risk, Lei says, an insurance company would need to ensure the algorithm is sufficiently insensitive to the behaviour of all customers in its database.
Privacy-first approach
Lei and colleagues Sentao Miao (McGill University) and Ruslan Momot (University of Michigan) have done extensive research into how firms can better approach privacy risks while still making data-driven personalized decisions.
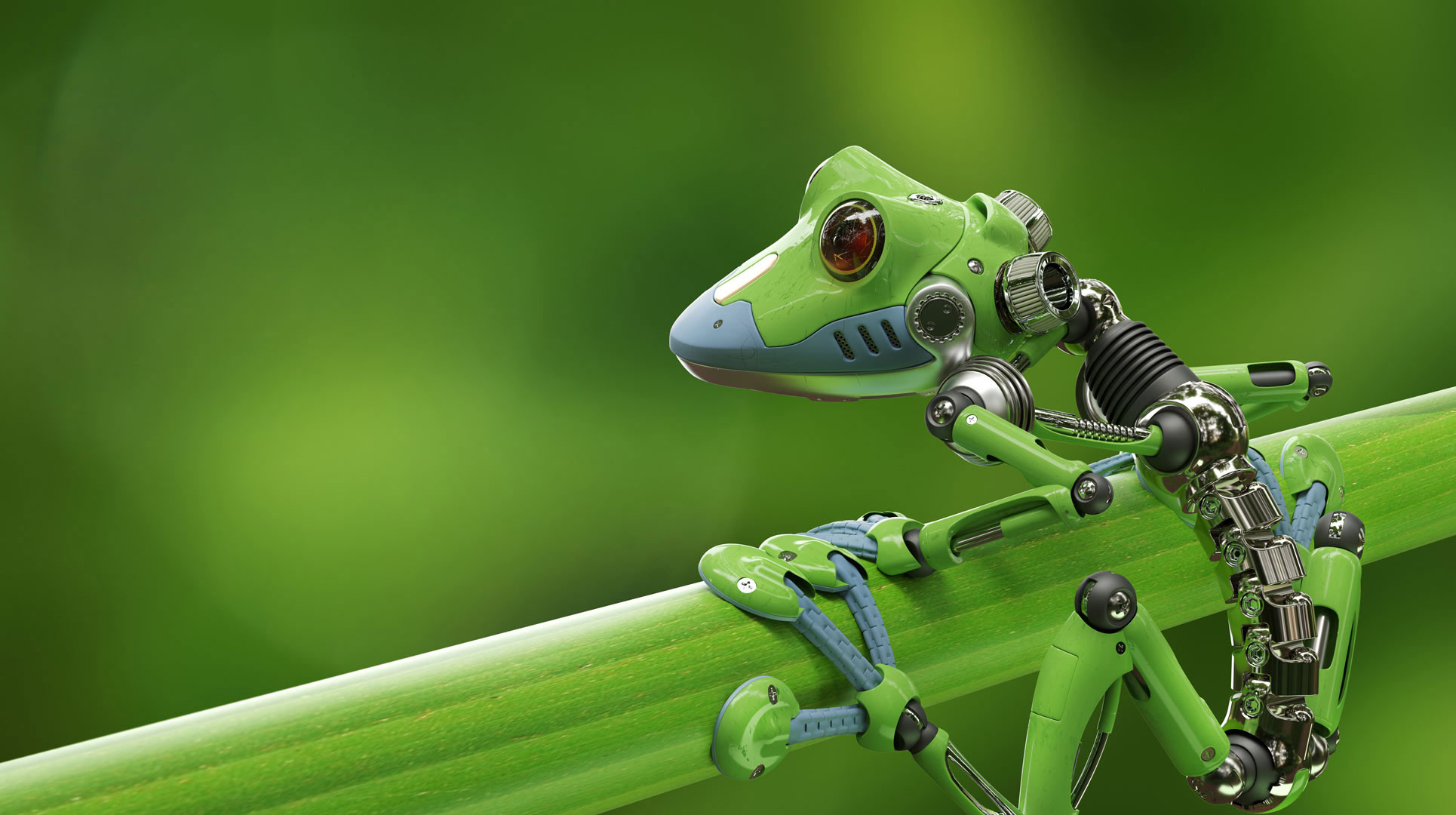
They found that the first step companies must take is to simply be aware of all kinds of potential privacy risks. When people talk about privacy risk, Lei says, “they tend to think that if they securely guard their data, and if they have very good IT security, then they’re safe. But this is not the case.”
Most importantly, Lei and his colleagues advise companies to build safeguards within their prescriptive analytics processes and algorithms. In their research, they suggest ways in which companies can rely on “differential privacy” — meaning that information such as product recommendations and pricing is not sensitive to individual customers’ data. This practice is already used by Apple, Google and Microsoft for different purposes.
They also suggest that companies inject uncertainties or “noise” into the decision-making process, making it more difficult to obtain personal customer data.
“In the example of the insurance company,” Lei explains, “if the algorithm does not always tweak the prices for customers who have a certain disease, or it only occasionally changes the price for customers who don’t have that disease, then it is harder for a hacker to infer the health condition of existing customers. The more uncertainties there are, the less privacy risk there is.”
In the extreme case that a firm charges prices uniformly at random to all customers, it is impossible for any hacker to infer any information about existing customers. Therefore, Lei says, the level of uncertainty added to the algorithm — and how uncertainty is added — directly determines the strength of privacy protection.
“This is particularly helpful for regulators,” he says, “since they can now quantify the strength of privacy protection, which makes it possible for them to pose quantifiable requirements to firms that use prescriptive analytics to make personalized decisions.”
Lei adds that regulators should also be counted on to help protect consumer privacy, and it should not be solely left to companies, whose objective, he says, “is to maximize profit.”
Despite these risks, Lei says prescriptive analytics is here to stay. While there is still a long way to go to ensure consumers’ privacy, he feels companies are becoming more aware of the importance of protecting personal privacy, as well as understanding that they need to align this form of analytics with societal values.
“As a society, I feel like there is more awareness of the privacy risks and more people are talking about it,” Lei says. “We want to harness the benefits of prescriptive analytics, but at the same time, make sure that we as consumers are not hurt.”