It’s Time for a Sober View of AI
Like rivers to the sea, biases will seep into AI tools and products. Businesses need to adopt new cultural lenses before it’s too late
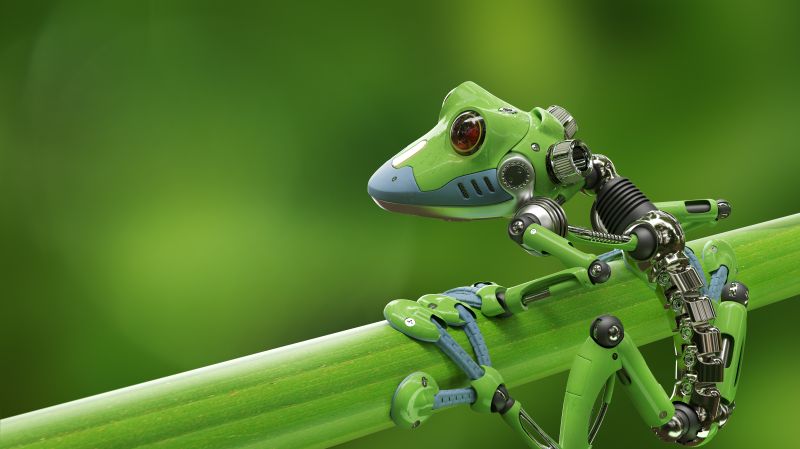
ChatGPT and similar buzzworthy tools driven by artificial intelligence (AI) are generating a good deal of enthusiasm in living rooms and boardrooms across the country. The biggest fans seem to be businesses in a wide variety of industries: they relish the opportunity to disrupt their markets or boost their productivity. These businesses are wise to closely follow developments in the AI space, but they must avoid being blinded by enthusiasm. Judging from AI rollouts to date, it’s clear the technology comes with baggage.
Consider data bias. This risk factor is behind many of the most worrisome AI failures. In the realm of law, AI tools have made biased predictions that falsely flagged Black and Latinx defendants as high-risk and white defendants as low-risk for committing future crimes. In human resource processes, AI tools have penalized resumés with information indicating female candidates while giving preference to those indicating male candidates. In health care, AI tools treat patients differently based on their demographic data.
Given these are not isolated cases, it should be clear by now that the uncritical use of data in AI can lead to unintentional biases with potentially negative consequences for individuals, organizations and society.
When organizational leaders misuse AI tools, it is often because they are blinded by a naturalistic view of data. Characterized by the popular metaphor “data is the new oil”, this view traps organizations in the belief that data are either objective and neutral representations of reality or merely reflect the world as it is.
This view is problematic. It ignores the fact that digital technologies are framed by social values and beliefs. Biases have been and will be inscribed in data that drive AI tools; in some instances, it is even mathematically inevitable.
Six pathways of bias
From our review of research on the use of technologies, my colleagues and I found six ways that biases — both overt and embedded — could sneak into data. These pathways vary widely:
Small group of experts. Studies have shown that expertise is often linked to power. Experts who possess related skills, educational backgrounds and research or work experience largely shape the design of digital technologies that eventually define how data should be collected, stored, analyzed and interpreted. Digital technologies reflect the worldviews of this comparatively small group of individuals.
Design with limited resources. When making design decisions, practitioners prioritize practical efficiency and effectiveness (such as time and hardware or energy costs) rather than the greater good. This pragmatic approach is particularly evident in “black box” AI techniques. Developers eagerly adopt black box AI because it outperforms traditional techniques, although they are unable to explain how it generates results or what potential risks could flow from its use.
Adaptation for limited cognitive capabilities. Humans tend to prefer data and data structures that fit established cognitive patterns, as they are easier to understand. Our cognitive frames often stick to known patterns and we struggle to adopt new ways of thinking. As a result, data must be presented in a clear and intuitive format. Although data presentation has evolved, the evolution has been closely tied to the subjective preferences of people in each era.
Theories embedded in digital technology. A long-standing viewpoint is that digital technologies are framed by popular theories from disciplines such as statistics, computer science, politics and psychology. Theories, however, are usually oversimplifications of a much more complex world. As theories shape the design of a digital technology, their use then profoundly shapes individual and organizational behaviours with the oversimplified worldview.
Uneven distribution of digital technology. The rise of data-driven decision-making is largely fuelled by advancements in sensor technology, computing power and storage capacity. But not all individuals, organizations or countries have access to such assets. The absence of a digital technology results in less represented social and material aspects of the world and limits the patterns that can be identified in the data.
Accumulated records. Records stored in databases are critical in determining what insights can be extracted; if data are not collected, no insights can be gained. Similarly, the way data are structured heavily influences the ability to recognize patterns in that data. And even if data records are available and well-structured, they could be trapped in outdated databases. The adage holds true: machine-learning models are only as good as the data they use.
Together, these social and technical factors suggest that even if every organization behaves with the intent for social good and every digital technology functions effectively, digital data will always arrive pre-framed and partial. It is theoretically and practically impossible for AI tools to be unbiased. Various logics will continue to be inscribed onto data — reinforced and magnified as AI emerges to shape the future of society.
Organizational culture change
It will be difficult to reshape political, economic and values-based norms around AI-driven decision-making once they are established. For that reason, organizations must be alert to the inevitable data bias from AI tools. Such reflective awareness of data bias requires specific cultural attitudes, three in particular:
Empathetic culture. Organizations with an empathetic culture care about human dignity and human rights. They develop and adopt AI tools with a deep understanding of how people are directly and indirectly influenced by their use. An empathetic culture motivates an organization to go beyond financial performance to work for the betterment of society.
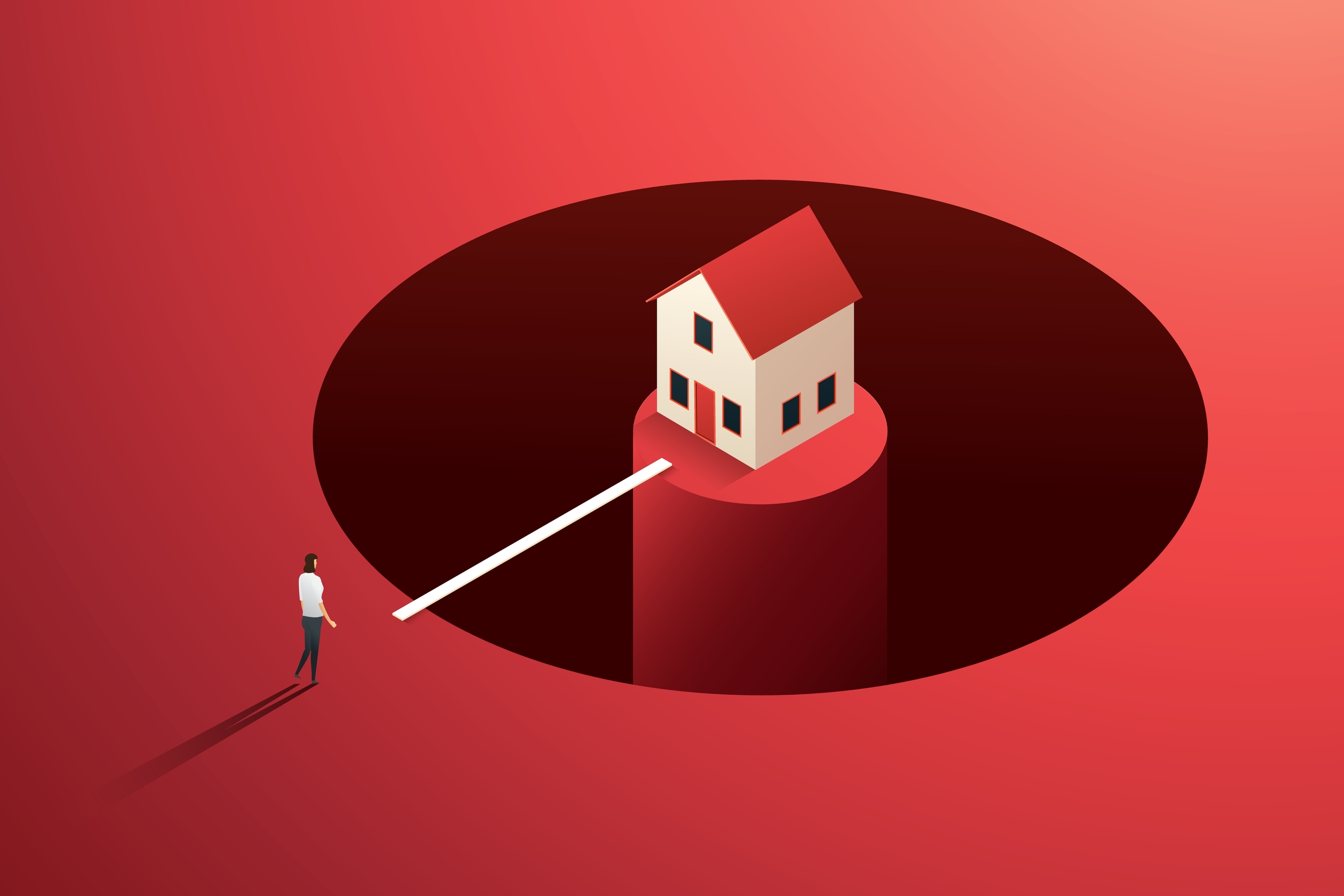
Holistic culture. Organizations with a holistic culture have a comprehensive understanding of what social and material factors shape the design decisions of their AI tool. Ultimately, digital data are partial surrogates of the world — they do not represent the full range of people, let alone the natural world. A holistic culture helps an organization remain attentive to how an AI application reflects widely diverse factors as well as their interrelationships.
Reflexive culture. Organizations with a culture of reflection critically evaluate their design decisions and use of AI. Being critical is an essential attitude for human-centred innovation: it helps organizations identify the limitations of existing technologies and go beyond them. Instead of assuming design decisions are neutral, organizations reflect on who made the decisions and how and why they were made.
New job description
Empathetic, holistic and reflexive cultures help organizations prevent the tragedy of data bias. Out of these cultures may emerge a new job position, an individual who can conduct “design forensics.”
We are already familiar with “algorithm forensics”; this is a procedure that analyzes the algorithmic trail for clues to understand any unintended negative consequence from an AI-based application. Complementing this rear-view approach, design forensics involves understanding and monitoring key decisions behind the design of an AI tool before it is operationalized. It determines why and how such decisions were made.
People filling this job would have three key skill sets corresponding to the three cultural attitudes: design thinking for being empathetic, systems thinking for being holistic and critical thinking for being reflexive.
As we fully enter the Age of AI, organizations must acknowledge that data records are not an impartial reflection of reality. They are creations of humans who intentionally or unintentionally bring their own biases into the process. AI tools may promise unprecedented productivity boosts and commercial opportunities, but they should be used with extreme caution, guided by new organizational cultures.
Gongtai Wang is an assistant professor in digital technology at Smith School of Business.