What Car Loans Can Teach Us About AI
Research on car dealerships shows how artificial intelligence can boost profits and help more people get a car
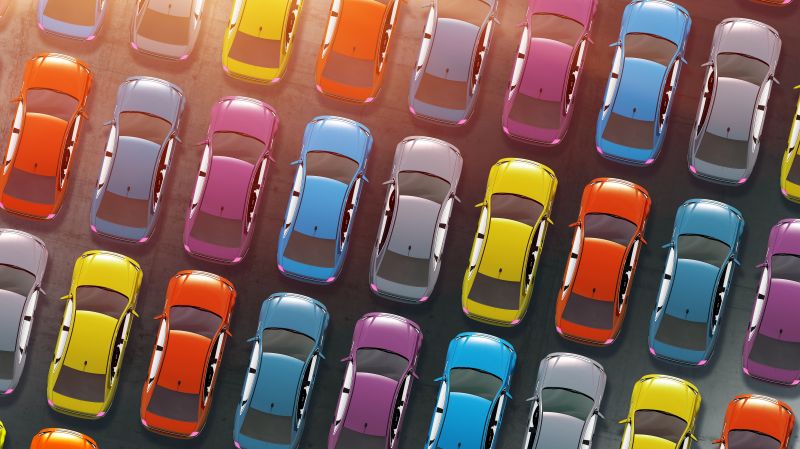
Artificial intelligence is changing the way businesses operate. More companies everywhere are deploying AI’s sophisticated tools to bolster efficiency and boost performance. A recent survey of AI adoption by IBM found that 42 per cent of organizations with over 1,000 employees now use artificial intelligence in their operations.
Yet, despite AI’s potential to improve business and society, some companies are still unsure whether to embrace it in their operations. Much debate remains around the technology’s risks. The IBM survey, for example, found nearly one in four (23 per cent) of respondents have ethical concerns about AI.
Can organizations capture the performance benefits of AI while mitigating the concerns that can arise with some AI models? That question is especially important for the financial industry and, more specifically, the decision of who gets a loan and who doesn’t.
“A lot of financial institutions have been wary of embracing AI, possibly due to concerns around transparency [think black box AI] and the potential for biased outcomes and customer backlash,” says Ceren Kolsarici, the Ian R. Friendly Fellow of Marketing at Smith School Business and director of the school’s Scotiabank Centre for Customer Analytics.
Beyond ethical considerations, Kolsarici notes that there are laws that prohibit the use of certain customer characteristics from being used in lending decisions. As a result, some industries continue to rely on traditional models to drive loan decisions.
“While AI bias is a well-reported phenomenon, I would argue that the use of AI based on well-understood and transparent machine learning and clean data that excludes demographics such as age, gender and race, has the potential to mitigate this risk as well as that in human decision-making,” says Kolsarici.
New research by Kolsarici and colleagues on how automotive dealerships offer loans to car buyers helps to make this point.
The right rate
At car dealerships, salespeople are often given a certain degree of pricing authority, the assumption being that they are in a better position to gauge a customer’s willingness to pay. When a consumer visits a dealership to buy a vehicle, the salesperson deploys their judgment, selecting from rate sheet options that balance the trade-off between receiving a high commission and closing the sale.
Does this traditional pricing model leave money on the table? Would an analytics-based approach be more profitable for dealers?
The study co-authored by Kolsarici, Mikhail Nediak (Smith School of Business) and Christopher Amaral (University of Bath) explores the effectiveness of the high-tech approach.
The researchers analyzed lending at car dealerships in Canada using loan application and approval data from a large North American financial institution. They then compared the profitability of the traditional model, in which salespeople select the interest rate for the loan, to a centralized analytics-driven model based on customer risk.
“Essentially we looked at whether analytics-based models were better at pricing loans for the average customer than salespeople and found that, as long as a company had access to rich data on their customers, AI models can pinpoint price sensitivity better than people can,” says Amaral.
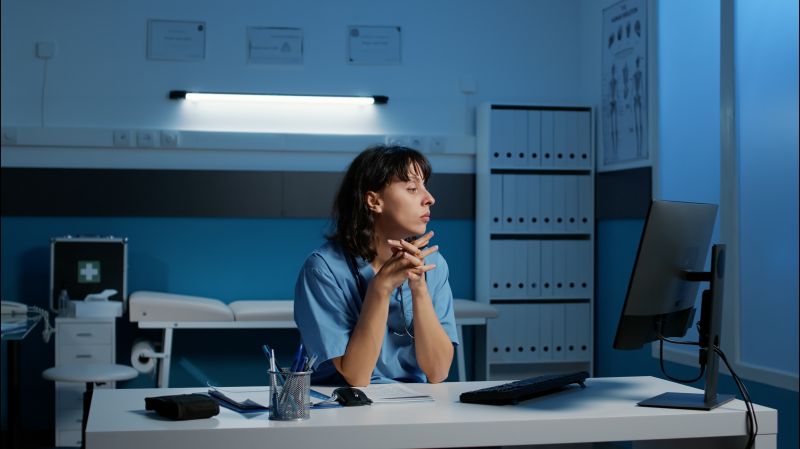
The study focused on factors such as consumer credit scores, loan-to-value ratios, types of vehicles financed and vehicle prices. The researchers then developed a model framework that optimized agent incentives and consumer prices by accounting for nuances in the indirect retail channel, such as competitor prices and commissions, and the interdependencies between the salesperson, firm and customer.
Wider applications
What did the researchers discover? Their results showed that using analytics-driven pricing based on customer risk combined with optimizing salesforce commissions could increase lender profits by 34 per cent.
The findings also revealed that the researchers’ model could open access to financing for consumers with traditionally low credit approval rates. The researchers note that these loans are more affordable than high-interest payday loans, which unfortunately are often a last resort for these consumers.
The modelling approach deployed in this study could also be used by companies in other indirect retail channels — such as travel agencies, real-estate brokerages and insurance services firms — to better understand the potential impact of data-driven analytics pricing models.
“Indirect lenders can use our model to understand how their pricing and commission decisions impact firm profitability and determine the profit lift associated with transitioning from pricing delegation to centralized pricing,” says Kolsarici.