How AI Can Help Make Wind Energy a Breeze
Wind turbines are expensive to build and maintain. Advanced technology can boost efficiency and ROI
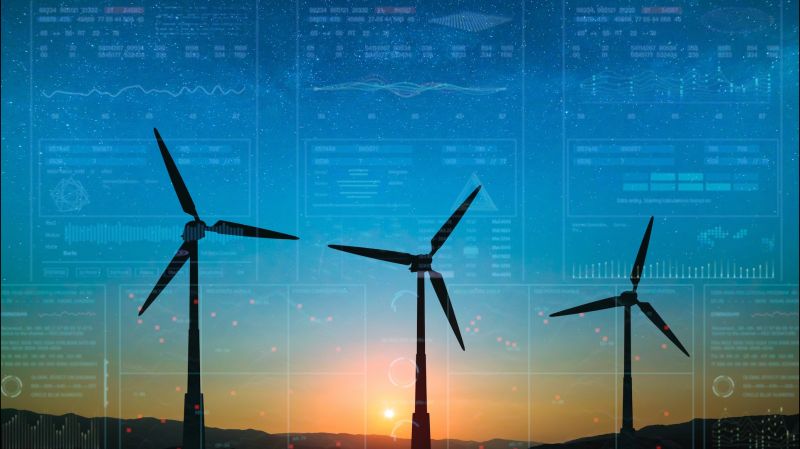
In June 2021, Canada passed the Net-Zero Emissions Accountability Act, committing the nation to zero emissions by 2050. To meet that target, we’ll need to reduce our reliance on fossil fuel energy. We still have a long way to go.
According to federal government data, more than 18 per cent of our electricity still comes from coal, gas and other fossil fuels. Only around seven per cent is generated by non-hydro renewables. The Canadian Climate Institute says that wind and solar energy capacity needs to grow by 95 per cent to meet the country’s net-zero commitment.
However, there’s some good news: The Canadian Renewable Energy Association reported a robust 11.2 per cent increase in renewable energy generation and storage capacity in 2023. That included a 74 per cent rise in wind-based utilities. Wind is now the dominant energy source on Prince Edward Island and is growing elsewhere, including in Alberta, Saskatchewan and Nova Scotia, provinces historically dependent on fossil fuels.
Yet wind projects are costly. For example, Canadian electricity provider TransAlta Corporation anticipated spending US$460 million to build 51 turbines at its White Rock wind project in Caddo County, Oklahoma, with an expected break-even point of more than 10 years.
Given such high costs, maximizing return on investment through increased productivity, reliability and cost-effectiveness is essential. One way to achieve better results is through artificial intelligence, analytics and machine learning. By employing these technologies in wind turbine systems, operators can address key maintenance challenges (including those with power converters and gearboxes) and optimize operations to deal with issues such as power curves, yaw misalignment, wake effect and grid integration. In this article, we’ll look at how analytics and AI can bolster wind turbine efficiency and returns in these key areas while helping to meet Canada’s net-zero goals.
When converters and gearboxes fail
About half of wind turbine downtime is due to electrical component failure in converters, generators and pitch control systems. These parts are expensive, accounting for 18 per cent of a turbine’s capital expenditure. When they fail, operators can lose thousands of dollars a day.
Power converters are particularly prone to failure and contribute significantly to downtime. Traditional ways to address such breakdowns rely on post-event analysis using control charts and statistical measurements. But these reactive approaches often lead to downtime before issues can be managed.
Advanced analytics can improve fault detection and prevention. Wind turbines are equipped with supervisory control and data acquisition (SCADA) systems that provide real-time data and remote-control capabilities. By training machine learning models on historical SCADA data, operators can automate the detection of regular and abnormal patterns caused by converter voltage failures. This predictive capability can enable timely responses, minimize the risk of extended outages, reduce hefty repair costs and optimize turbine productivity.
A wind turbine’s gearbox is part of the system that converts the rotational speed of the “wind wheel” into electricity. Gearboxes often operate under extreme conditions, including high temperatures and varying velocities. Such demands can lead to higher fault rates and the risk of irreversible turbine damage.
Using SCADA-collected data, operators can monitor gearbox performance indicators like temperature and vibration. Through convex optimizations (e.g., linear programming, quadratic programming and least squares), they can predict gearbox reliability by comparing these patterns to a large set of known patterns and pinpointing problematic conditions. This enables consistent predictive maintenance and fault detection programs.
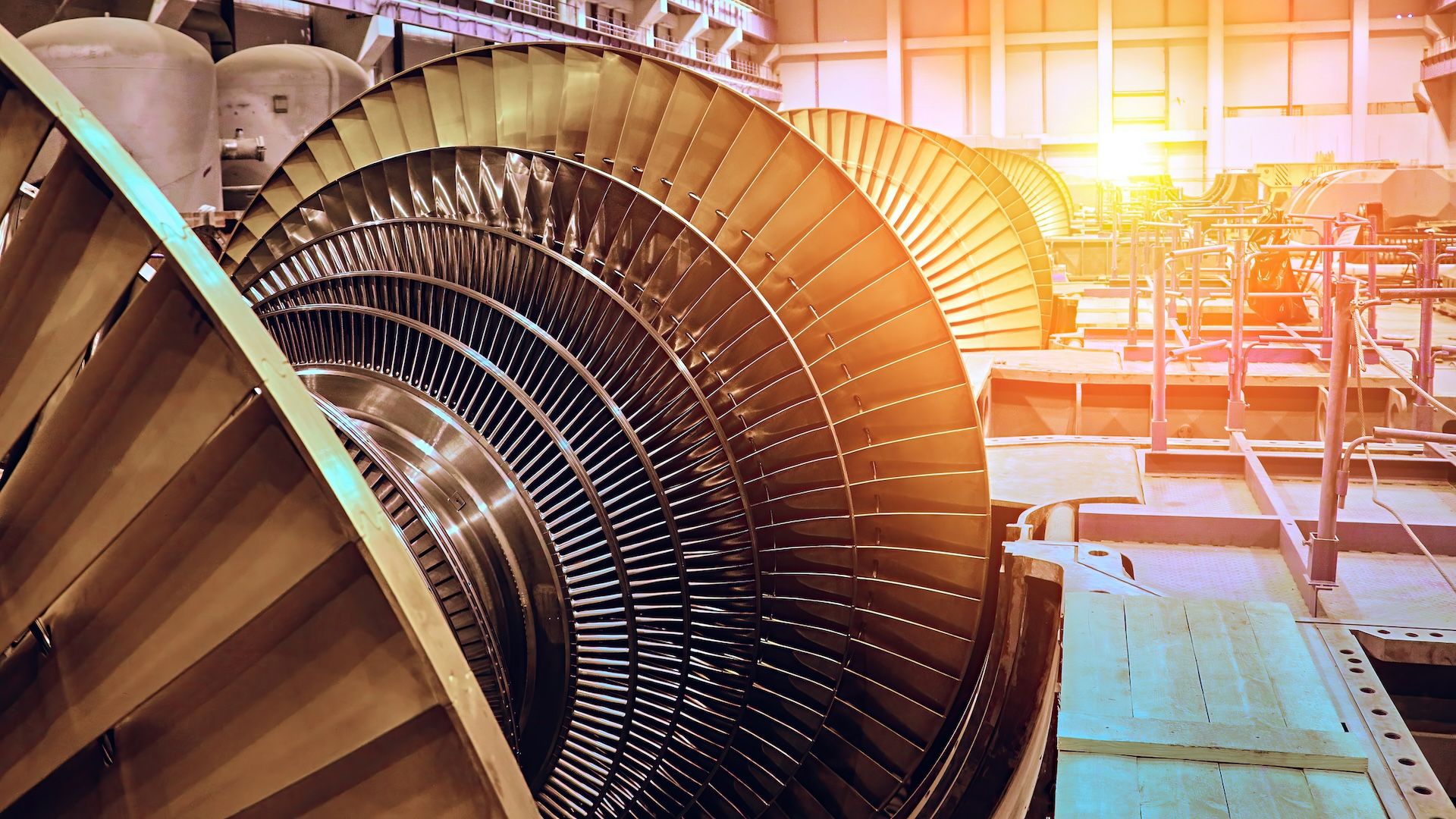
Modelling power curves
A wind turbine’s power curve reflects the relationship between wind speed and power output, serving as a tool to assess and monitor performance. But traditional power curve modelling techniques can be simplistic and lead to prediction errors.
Machine learning can offer a roadmap to extract the maximum possible energy from the wind. Algorithms can analyze historical data to identify patterns and anomalies in turbine performance and improve the turbine’s output in real time. Predictive models can forecast wind conditions and adjust the turbine's settings to maximize power generation while minimizing wear and tear. This can improve energy generation under varying wind conditions.
In search of yaw alignment
Yaw alignment refers to the position of a turbine rotor relative to wind direction. The yaw drive rotates the nacelle (the enclosure atop the turbine containing the gearbox, shafts, generator and brake) to align with changing wind directions. Yaw misalignment can lead to significant power losses and increased mechanical stress. Proper alignment, on the other hand, improves energy production and revenue.
One common way to maintain yaw alignment is through LiDAR (light detection and ranging technology), which calculates wind speed by measuring the Doppler shift caused by the motion of air particles. Mounted on the nacelle, LiDAR sensors allow operators to anticipate wind speed and direction, adjusting yaw alignment to increase power output and reduce wear.
But LiDAR is expensive. Machine learning can help by using real-time data to predict wind approaches, reducing the number of LiDAR systems needed on a wind farm while maintaining accuracy. This approach cuts costs and enhances turbine efficiency through proper alignment with incoming wind.
Watching for the wake effect
The wake effect occurs as wind passes through a turbine, reducing downstream wind speeds and increasing turbulence. As the turbulent air travels, it spreads to other turbines and nearby wind farms. This can stress turbine components and reduce productivity across installations.
Managing the wake effect is possible, but it is also complex and requires sophisticated modelling. Here again, AI can play a role. Machine learning models rooted in statistical-driven methodologies can offer a more accessible alternative to currently used physics-based simulations. Deep neural networks, such as CNN-LSTM, can model wake dynamics and predict their impact on downstream turbines — capturing the nuances of time series data and revealing nonlinear relationships that might not be captured in a physics-based model.
With all this data in hand, an advanced control strategy, like dynamic wake steering, can adjust yaw alignment of upstream turbines to deflect wakes away from downstream turbines. Such a strategy can reduce energy losses and increase efficiency across the entire wind farm.
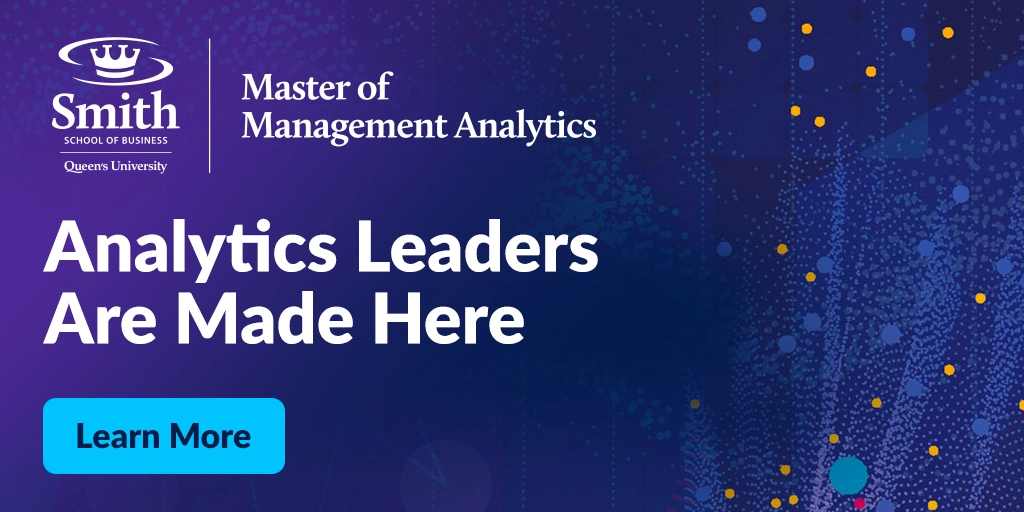
Grid intentions
Wind is not a constant resource. As a result, electricity grid operators must rely on alternate reserve generation, often from fossil fuels, to fill the gaps on non-windy days.
Analytics and AI can offer support. Analytics can predict weather conditions and, consequently, wind power output, helping operators plan and allocate reserve power efficiently. For wind farms with energy storage systems, analytics can be combined with usage estimates to determine if additional capacity will be required from another portion of the grid.
Machine learning can further support grid integration by alleviating many of the maintenance challenges as described earlier in this article. After all, increasing the reliability of wind turbines will decrease unscheduled outages. This makes the entire grid more reliable since wind turbine failure is less likely to occur. Scheduled maintenance outages can then be planned to coincide with sub-optimal weather to reduce production loss.
Powering our renewable future
Today, governments and corporations are being asked to build a sustainable future. Wind energy can play a crucial role in that effort. Yet simply adding more wind turbines is not enough.
Operators must use machine learning to protect their investments by reducing maintenance costs, minimizing downtime and preventing component failures. Additionally, AI algorithms can maximize turbine production and improve performance.
Introducing these technologies into new and existing wind farms is a smart move. Canada’s grid will require such innovations to meet our net-zero emissions goals and provide a reliable and cost-effective source of clean electricity for future Canadians.
This article was researched and written by a team of Master of Management Analytics students at Smith School of Business: Alex Hardie, Reid Foose, Laura Wang, Remilekun Osibogun, Wish Bakshi, Dori Small and Ricky Yuen.