Putting AI Ethics into Practice
Most regulators have yet to release formal AI ethics regulations. Here’s how firms can successfully implement their own guidelines
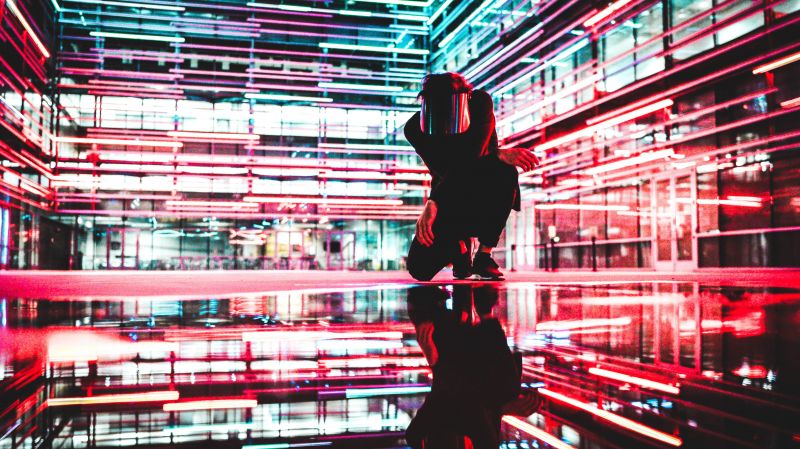
Wouldn’t it be great if using brand new technology could be like playing a board game? You open the box and everything needed is there—certainly all the physical pieces but also the rules of the game and what constitutes fair play.
Emerging technology doesn’t work like that. Artificial Intelligence is a recent example: the first wave is already upending how organizations operate and serve customers and stakeholders—and no one knows half of what this set of technologies will lead to. In financial services, an eager AI early adopter, there are tales of misused data, biased outcomes and other ethical red flags.
But where are the rules defining how AI is to be applied, enforceable guidance that says you can go to this line but not beyond? In North America, at least, the development of emerging technologies is running ahead of our ability to regulate them. It’s like playing Catan and deciding to re-roll until you score enough lumber.
Absent external regulations, some firms try to self-regulate by setting up oversight boards or, more likely, drafting AI principles or ethical guidelines. These guidelines are high-level normative statements on how to use data and develop AI products ethically. They are part of the long lineage of business codes, says Stephanie Kelley, BCom'12, MMA'17, PhD'22, a PhD candidate in management analytics at Smith School of Business who is studying how AI principles are being adopted.
Rather than applying to all employees, as is the case with business codes of conduct, AI principles are designed only for employees who use or work with AI tools.
Principles are not enough
Kelley’s study focused on the financial sector, perhaps the most aggressive early adopters of AI outside of technology firms. She conducted in-depth interviews with 49 people working directly with AI at 24 banks across 11 countries.
Her big takeaway is that AI principles alone are not enough to keep organizations from ethical blunders. “The findings from the study show that you need to have lots of targeted initiatives that help address the organizational challenges of using AI,” says Kelley.
From her study, Kelley identified 11 components that can impact the effective adoption of AI principles, seven of which apply to general business codes. These are factors such as communicating the principles, measuring performance and training staff.
“It was the four last ones—the accompanying technical processes, sufficient technical infrastructure, organizational structure and interdisciplinary approach—that were really surprising,” she says.
Technical processes refer to technical manuals, checklists or ethics impact assessments that translate the principles into a framework that can be implemented by data scientists and data engineers.
Technical infrastructure involves an inventory of every active AI project in the organization as well as data and system compatibility. It’s virtually impossible to implement AI principles if data is missing or computer systems are inaccessible.
A centralized organizational structure is also likely better for assigning responsibility, completing the AI inventory and enforcing the principles. And an interdisciplinary approach draws insights from people within the organization and outside in a wide range of roles, from AI practitioners, technologists, lawyers and senior managers to academics, ethicists, AI vendors and even regulators.
Off-the-shelf guidelines
These factors make intuitive sense, but they are challenging to enact. Even well-intentioned organizations—those that aren’t using AI principles for ethics washing or to evade regulation—struggle to make the principles work.
“It's a continual challenge,” she says. “When I interviewed them, people said that AI principles were too high level and not detailed enough. But they felt they had to draw a line in the sand to say, We’re going to try and uphold these principles even if we’re not yet sure how we’re going to do it.”
Given these challenges, some organizations are deciding instead to adapt ethical guidelines developed by non-governmental bodies or standard-setting agencies that reflect feedback from a broader range of stakeholders.
The European Union has gone one step further and released the Artificial Intelligence Act, a formal AI ethics regulation that includes principles. The act takes a risk-based approach. It identifies different uses and environments in which AI can be used, and then offers regulatory guidance based on the risk level of the particular AI technology.
With the EU putting its stake in the ground, will there be momentum for higher ethical standards governing AI use in the future? Kelley says it is a contested issue. “Some people, like those in the EU, believe there’s going to be a race to the top,” she says. “This idea that if you give a good example of how you should behave, it will become a competitive advantage or point of differentiation for businesses. On the other side, some believe it’s going to be a race to the bottom, and that these ethical issues are not important enough to outweigh the uses of AI that may be somewhat unethical but will make a lot of money.”
If you ask people who work directly with AI, most are betting on the race to the bottom. That’s what Pew Research found in a survey of more than 600 technology innovators, developers, business and policy leaders and researchers. When they were asked to consider the state of AI in 2030, 68 per cent said they thought that most AI systems would not employ ethical principles focused primarily on the public good.
Their reasoning is based on power and money: Big corporations and governments using AI are focused on profit-seeking and social control; there’s little consensus on what ethical AI looks like; and AI applications are already operating in “black box” systems that are difficult, if not impossible, to dissect.
As Kelley found in the financial services sector, the value of AI ethical principles rests on the ability of firms to develop the technical manuals and checklists, extensive inventory of data assets, compatible systems and the right structure to enforce the principles. Without that, the data scientists can do little to translate high-level guidance into front-line vigilance against harmful and unintended consequences of AI.
The choice is clear: Organizations can settle for toothless AI ethics guidelines and risk lulling themselves into a false sense of security. Or they can get serious about operationalizing AI principles that drive profitable and ethical AI applications.
Photo: Unsplash/Drew Dizzy Graham