Customer Wait Times Are No Idle Concern
Managerial tweaks can reduce lineups, but they can also backfire
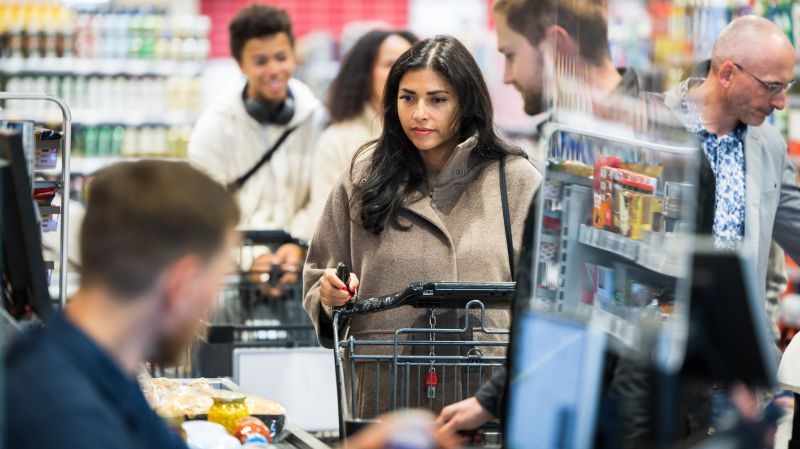
We’ve all been there: it’s 5 p.m., you’re hungry and waiting in a grocery store lineup that is not moving. Your mind is in overdrive. Should I move to a different line? Why isn’t the cashier working faster? When will another lane open? Should I abandon my cart and walk out?
Raga Gopalakrishnan knows all these questions well. He grew up in India, a country famous for its queues. “Whether it’s a doctor’s office, ATMs, polling stations, airports, traffic — there are so many lines you have to wait in,” says Gopalakrishnan with a smile. “And waiting there myself, I got interested in watching what people would do.”
That light hearted fascination has turned into a serious academic passion for Gopalakrishnan, an assistant professor of operations management at Smith School of Business. Gopalakrishnan’s research partly focuses on how customers, servers and managers deal with lines — and what might be done to reduce all that idling.
The implications can be huge. Yes, queueing can make people walk out of a store or rob Americans of 37 billion hours each year, but it can also have serious health impacts. One recent study even showed that long patient waiting lists were associated with poor patient outcomes.
Gopalakrishnan’s recent study looks at what managers can do to reduce wait times. He and his co-authors developed a mathematical model that suggests how managerial decisions about staffing and payment could have a big effect on employee work speed that can lead to long queues.
Questioning the queue
Gopalakrishnan and his colleagues — Yueyang Zhong and Amy Ward of the University of Chicago Booth School of Business — are not the only researchers interested in lineups. Their area of focus, known as queueing theory, is a burgeoning field that focuses on the mathematical study of waiting lines.
Some queueing theory research looks at the customer experience, such as why customers decide to stay in or leave a line. Other studies investigate the employee angle, particularly work speeds in response to queues and the policies governing their management.
A lot of queueing research, particularly in the 20th century, has looked at automated systems such as manufacturing sites and data centres. For good reason, says Gopalakrishnan. Some of these systems demand significant energy resources that can be reduced by tweaking the queueing design and scheduling policies.
But there is an increasing need for queueing theory to evolve to account for the boom over the last two decades in the service economy and the shift towards customer-centric services.
“That evolution is happening, but it’s been somewhat slow,” says Gopalakrishnan. “It’s also been lopsided on the customer side. But I’ve been more focused on the server side: What kind of models can capture server behaviour? And how can those behaviours be integrated with queueing models to see how that affects the optimal decisions by management?”
Limits to incentives
Those questions are part of what Gopalakrishnan looked at in his most recent study. It began with the assumption that service employees can work faster or slower in response to the service environment, thereby affecting customer wait times, among other effects.
But given that this study is based on mathematical modelling rather than real-world data, it has a few other assumptions baked in. One, for example, is that employees are self-interested and value their break time and work time in a specific way. Another is the effort people put into working faster versus working slower.
Given these assumptions — which are partly based on past empirical research — the model came up with several intriguing findings. Gopalakrishnan groups them into several themes.
One is that there are limits to incentives. “For instance, we find that if managers keep decreasing the number of staff, yes, it can encourage servers to work faster at first, but there is a limit to that compensatory response,” says Gopalakrishnan. And when servers are pushed too hard, they may slow down and even rebel.
Payments can have a similar effect. Managers can pay staff more money to incentivize them to speed up. But eventually workers will hit a physical limit and won’t be able to work faster.
A second theme is that there are counterintuitive ways in which incentives affect employees. A prime example is that increasing payment for each customer or job that is served can encourage employees to work faster.
“You have to be careful,” says Gopalakrishnan, “because your high-performing servers will work faster, but your low-performing servers that opt for a different trade-off between work time and break time might slow down even more and become worse. This may be because they can now afford to serve fewer customers without significantly impacting their total monetary compensation.”
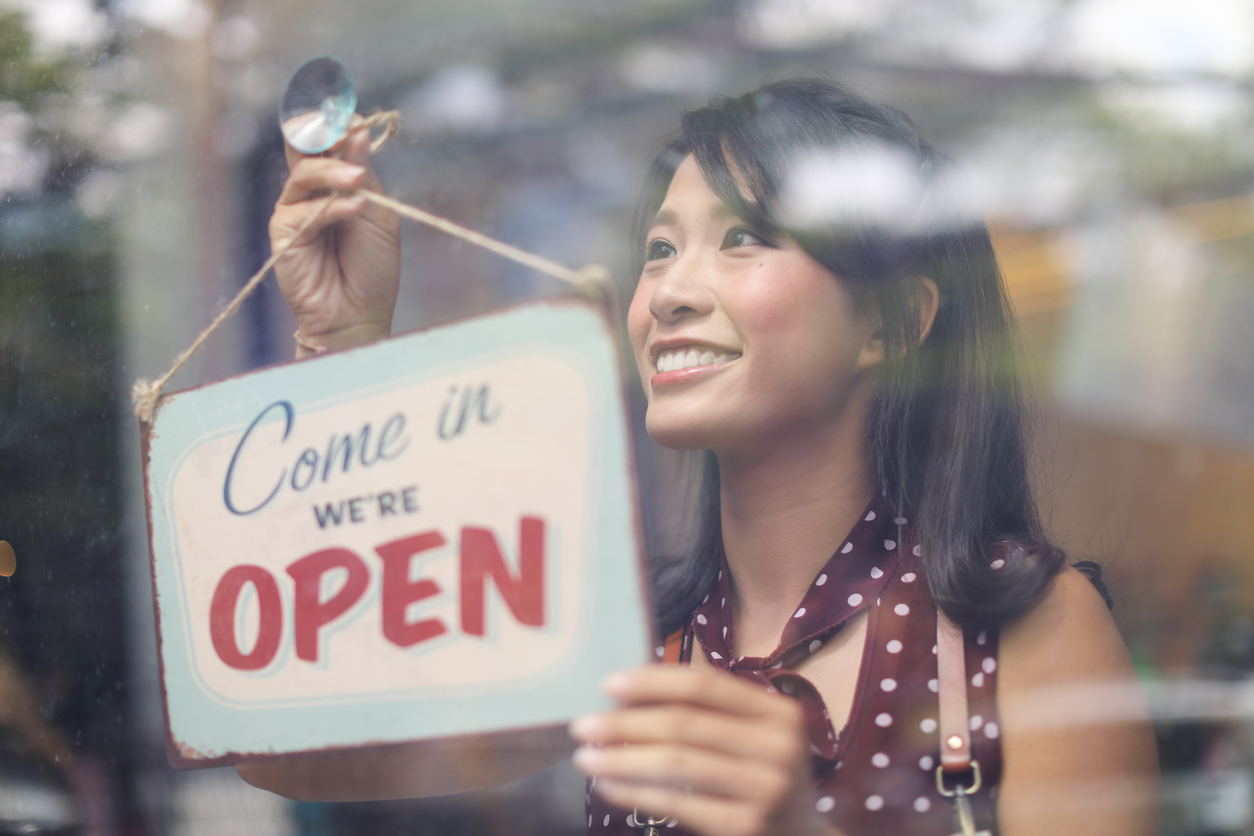
In short, managers must think strategically about the interplay between systemic and monetary incentives, says Gopalakrishnan. “You can tweak systemic policies that don’t cost money, like improving how your queues are structured or how waiting customers are matched to available employees,” he says. “But at what point do you realize that isn’t enough and you need to spend money to hire more servers, for example?”
Gopalakrishnan is quick to point out, however, that his study does not offer precise recommendations to service system managers seeking to improve their queueing policies. Rather, it’s part of a learning loop of investigations between theoretical researchers — Gopalakrishnan’s camp — and empirical researchers who could validate the results through lab or field experiments.
Still, managers should pay attention to these themes, he says. “At least keep them in mind so that they’re not surprised if employees respond to their decisions in some unexpected way. If they do that, they’ll be equipped with the tools to recognize quickly why that could be happening and think about possible ways of mitigating that in the future.”