A Way to Uncover HR's Hidden Insights
Natural language processing is changing the way human resources departments work
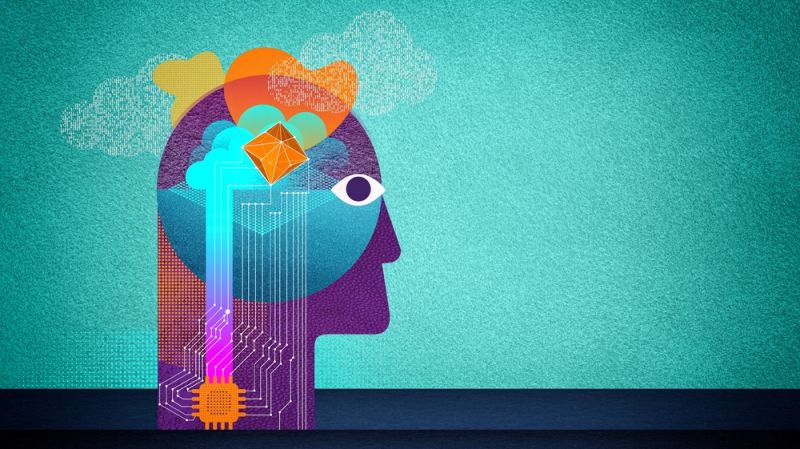
If there’s one thing human resources departments have enough of, it’s documents filled with words. Years’ worth of employee surveys, performance reviews, resumés, onboarding files and more. Historically, little has been done with these mountains of information.
For example, how many times have performance reviews been read at year-end and then filed away and forgotten? How often have surveys been created to ask employees’ opinions, only to have the open-ended comments disregarded because it takes too long to read them? How often have recruiters tried to sort through hundreds of resumés to find the perfect candidate for an open position?
Natural Language Processing (NLP) is changing all that. It’s allowing HR departments to automate and optimize the process of deriving insights from all the words and data in these documents.
What is NLP?
Too often, terms like artificial intelligence (AI), analytics and natural language processing are used interchangeably or as biz-speak. It’s essential to understand the differences in these terms before we explore how they can be applied to human resources.
- Natural Language Processing (NLP) is a subset of artificial intelligence and provides tools to help us understand and extract meaning from text. The goal of AI is to make machines think and act rationally like humans.
- Text analytics is the process of deriving insights from text. Analytics is what humans do, while AI is what computers do.
NLP comes in many forms. Most people are familiar with virtual assistants like Apple’s Siri or Amazon’s Alexa. Both use NLP to understand voice commands. Chatbots are another form of NLP. They interact with people via text exchange and can be used for anything from providing product information to placing orders.
There are many different practice areas in NLP. For this article, we’ll focus on three—sentiment analysis, document classification and topic modelling—and show how they can be used in human resources.
Sentiment analysis
Sentiment analysis is sometimes called opinion mining or emotion AI. It is the process of automatically determining the emotion of a text document. For example, does the document contain anger, fear or joy? Sentiment analysis can be achieved through many different methods, including supervised and unsupervised machine learning.
Commonly, sentiment analysis can determine the “polarity” of a document. Is it positive, negative or neutral? Extracting emotion from a document can be more valuable than simply knowing its polarity. However, emotion extraction is a complicated process that is more susceptible to errors.
Sentiment analysis is often used to determine the customer’s voice and understand what customers feel, think, need and want. In HR, sentiment analysis can be a helpful tool to assess employee emotions. It can be applied to open-ended comments in employee surveys, including onboarding, engagement and exit surveys.
For example, sentiment analysis of an onboarding survey can let an organization know whether new employees feel optimistic about joining the company and whether they are set up for success. Organizations can go as far as monitoring employee sentiment by analyzing company emails to determine employees’ overall happiness and satisfaction. Sentiment analysis can even help predict which employees are at risk of leaving.
Document classification
Document classification follows the same process as the machine learning technique of classification, a type of supervised machine learning. The main difference is that the features used in the classification algorithm are text extracted from documents. It is important to note that in document classification, features extracted from text are often used to supplement, rather than replace, other structured features that may be available in the data.
One of the most common document classification applications is the automatic detection of spam emails. In HR, document classification can triage and route job applications to the recruiter responsible for hiring for that particular role. Document classification can also provide additional insights to enrich employee risk-of-exit predictions by classifying employee emails, texts or calls.
Topic modelling
Another valuable NLP technique is topic modelling, a form of unsupervised machine learning. Topic modelling is the process of extracting high-level themes (a.k.a. topics) from a document. Topics are not predefined but are automatically determined by the algorithm.
Through topic modelling, we can quickly and automatically (and without training data) generate central themes from hundreds of thousands of documents. Topic modelling algorithms rely on the statistical word counts in documents and use machine learning to determine the most prevalent topics. The idea behind topic modelling is that words occurring frequently in documents will belong to the same topic.
Topic models have many business purposes and are helpful when summarizing large amounts of text. In HR, a performance review that contains many open-ended text responses can be analyzed and its central themes uncovered. So instead of reading hundreds or even thousands of reviews, we can simply read the topics to better understand the most prevalent themes and determine which ones have positive, negative or neutral sentiments.
These are a few examples of how NLP will change the way human resources works. There is so much opportunity within HR today and it’s exciting to see how people will use these innovations and new technologies to help organizations make better decisions.
Heidi Klotz is director, total rewards and HR analytics at Kruger Inc. in Montreal. She holds a Master of Management Analytics degree from Smith School of Business.