The Route to Trusted AI
What the financial services sector can teach us about preparing for the age of artificial intelligence
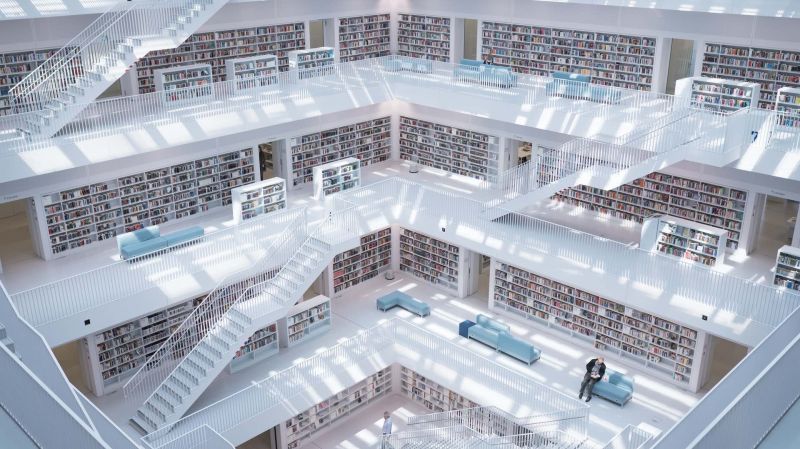
“We weren’t even allowed to say artificial intelligence until six months ago, when our CEO used the term by accident. Now we’re on a journey to figure out what AI means for us.”
This surprising admission was shared by an executive from one of Canada’s major banks at an industry event in late 2019. Now, less than two years later, you can’t talk about financial services without mentioning the impact of artificial intelligence on operations and customer experience. Each bank is on its own journey. Some are buying niche technology leaders, others establishing centres of excellence or partnering with universities. The bank that wouldn’t even utter “AI”? It spent more than $150 million last year exploring its options.
Canada’s big banks are certainly well placed. AI requires data—lots of data—to be fed into machine-learning models. Banks are to data what Saudi Arabia is to oil. With credit card, mortgage and other financial transactions in hand, the typical bank likely knows more than 25,000 things on each of its clients.
Machine learning can refine these vast reserves of data into new customer services. Banks are already piloting or rolling out AI and machine-learning applications. For example: a forecasting tool for car dealers to predict demand for vehicle purchases based on customer data; a ranking system that identifies which clients are most at risk of leaving, and the best ways to retain them; and a finance and budgeting tool that offers clients personalized advice based on their spending patterns.
But the banks have also come to realize that, like it or not, AI is a journey they will have to take with one another—at least part of the way. Two strategic insights explain why: One, regulation is coming and banks had better get ahead of the wave and align on key issues. And two, in AI, where trust is the price of admission, banks are only as good as the weakest among them. A misstep by one bank that undermines trust in AI hurts them all.
“In Canada’s financial services sector, the products are quite similar,” says Stephanie Kelley, a PhD candidate in analytics at Smith School of Business. “So if one bank is found to be using AI unethically, the thought is that the entire industry would suffer.”
Playbook for trusted data
It is no surprise, then, that financial services firms are trying to sort out AI ethics issues together, largely through a neutral third party, the IEEE (Institute of Electrical and Electronics Engineers), a global technical professional association that sets technology standards. As a measure of their progress, the IEEE has just published the Trusted Data and Artificial Intelligence Systems (AIS) Playbook for Financial Services, intended to help move AI ethics principles into practice.
The playbook, which Kelley co-authored, incorporates insights from more than 50 technical, legal, analytics and risk management experts from Canada, the U.S., U.K. and Southeast Asia. It offers more than just a clearinghouse of resources and a guide to the global regulatory landscape. Through 20 high-value AI use cases, the playbook shows how concerns such as human oversight, transparency, non-discrimination, societal well-being and accountability are being handled in the real world. And it includes a road map and anonymous companion survey for firms to determine their stage of AI and trusted-data readiness.
Where will this road map ultimately lead banks? Kelley says that today’s banking model could evolve from transaction-focused credit institutions into “service-based digital platforms that place relationships front and centre.” Such AI-based platforms would be optimized to create and sustain trusted digital relationships. Think of the “data-driven bank” serving the “data-enabled customer,” she says.
Building blocks
According to research Kelley conducted, organizations that successfully connect trusted data to customer value have three building blocks in common: people, process and technology. Not surprisingly, the “people” building block touches on corporate culture: a strong data and AI climate; leadership buy-in based on a clear data and AI strategy; and enterprise-wide education on trusted data and AI.
The “process” building block involves data and AI ethics governance tools such as impact assessments, key performance indicators and, of late, standards and certifications (including several from IEEE). And the technology building block is all about a consistent scaling process centred on machine learning and DataOps (data operations). This requires a resolve to ensure each AI project is conducted with trusted data and AI in mind.
While the IEEE playbook offers a clear path to ethically sound AI initiatives, travelling down that path will be a challenge. As Kelley notes, while the regulatory framework is still being developed, firms can expect banks and other financial institutions to face new legal requirements around transparency and auditability. For example, the proposed Artificial Intelligence Act, just released by the European Commission, will impose strict requirements for several high-risk AI banking applications, such as credit lending models.
Fulfilling this requirement will demand, among other things, explainability—helping clients or regulators understand how an AI system arrived at a conclusion. This a tall task considering the complex ways data is processed. Explainability demands a level of transparency that is considerably higher than what is often practised today. Regulators are still figuring out how to proceed, but some banks aren’t waiting. One bank, for example, has committed to storing all decisions that are made via machine learning.
The banks may be well-intentioned but they should be careful of what they promise. While there are benefits to greater transparency—the mitigation of bias among them—there are significant risks as well. Researchers warn that releasing information about models and algorithms may make AI more vulnerable to attacks. It has been shown that entire algorithms can be stolen based on their explanations alone and that two popular techniques used to explain certain types of algorithms can be hacked.
While there is a sense of urgency to iron out the requirements for trusted data, there’s an equal urgency to push AI deeper into operations. A major challenge for banks is to cut the time it takes to get AI models into production and actual use. If the time-to-market cycle cannot be trimmed, the benefits of AI will diminish.
Kelley sees this natural tension between ethics and execution as healthy—they are both about delivering business value. “If you don’t have trusted data and AI as the foundation, you won’t be able to deliver on the ultimate value of those applications,” says Kelley. “You may be able to implement AI, but at some point you’ll hit a roadblock around the ethics. You’ll be risking much more in your business than not having that application in place.”
For more information on trusted data in financial services, download the IEEE playbook, take the 20-minute survey on AI readiness or contact the playbook team.
Stephanie Kelley’s PhD research at Smith School of Business has been supervised by Yuri Levin, Professor of Management Analytics (on leave) and Professor David Saunders.