Curriculum
A consistent refrain from employers is the growing need for managers with a solid understanding of both finance and the impact of data science and machine learning. With the rapid advance of fintech and other developments, this demand continues to grow. Smith’s MFIT program will fill this skills gap.
Essential to survival in the digital age is an organization’s ability to discover new technology-enabled opportunities and deliver solutions that realize benefits. As technology becomes more pervasive, the intersection between IT and traditional business functions has blurred ushering in new practices in digital strategy, design, development, and implementation. This course introduces students to modern practices in designing digital innovation that incorporates foundational concepts inherent in design and systems thinking. By taking this course, students will develop the critical thinking skills needed to reimagine business models and deliver solutions that make an impact.
This course will explore why a range of fintech innovations (e.g., token-based economy) have struggled to prove their viability. Several reasons will be discussed including digital shifts being approached as technology solutions (i.e., cryptocurrencies) as opposed to a digital business transformation initiatives, and the challenges of integrating department-specific digital initiatives so that new products and services give stakeholders (i.e., customers, employees) what they want and need. This course will culminate in a “Pitch Day” for the MFIT students.
In this course you will learn how technology has changed financial markets and institutions function. You will learn key concepts for evaluating electronic market quality like liquidity and price discovery. You will also learn how trading algorithms work, how machine learning and big data are being used and about innovations like high frequency trading and dark markets. All of these aspects are changing the skills required to be successful in digital capital markets. We will also cover how trading, clearing and settlement has changed and is changing, the skills required to be successful and what the future may bring.
Gone are the days of the portfolio managers pouring over the annual reports of companies to make investment decisions. Today, algorithms and computers are independently making investment decisions across markets and asset classes. Data now takes the front seat in the investment decision making process. POS sales data, satellite images, social media posts, or search queries are now equally important when making an invest, or divest decision. This has changed the skills required of portfolio managers and how the market for advice works, how clients access expertise and the role of financial professionals in the investments industry. In this class, we will cover how data science and machine learning algorithms are changing how fundamental research is performed. We will also cover the recent rise of robo-advisors, and automated advice, and understand the role of other types of wealth-technology.
Data is the new oil. Financial data may be the new gold. Securing this data and ensuring the privacy of clients’ data is critical to deriving value from this data. This course will explore topics on financial cyber-security, fraud, and money-laundering. The course will explore and explain how hashing in distributed ledger technologies works, how the public-private key infrastructure ensures identities, and why non-technical security measures are important. We will also explore ways to leverage security and privacy to generate new insights via federated learning!
The course will establish a foundation of statistical modelling techniques to be immediately useful for analysis and to provide a foundation for more advanced material studied throughout the program. Topics will include data types, random numbers, probability models, hypothesis testing and statistical inference, and a thorough grounding in simple and multiple regression. The course is designed to ensure that all students, regardless of background or experience, are proficient in the use and application of a variety of statistical methods.
This course will focus on the data management techniques frequently used as a precursor to analysis with ‘real world data’. Topics include database structures, SQL, data cleaning, merging and filtering, detecting and correcting errors. The course will also cover the application of visualization to developing and telling stories with data with data visualization techniques.
The course will combine three key elements: analytics techniques, business applications, and basic coding/programming (in R, one of the leading open-source tools for analyzing data that you will be able to use in your jobs.) The emphasis will be not on the technicalities or theory, but rather on applications to various business cases. Basic familiarity with R is required, but for most classes you will receive a starter code, by running and modifying which you will learn analytics techniques and coding principles, and which you will also be able to use in your jobs. Because of that, much of the course will be in a form of a "hands-on" workshop; students are be expected to bring your laptop to class (with all the necessary software tools installed) and actively participate in the learning process.
The course will cover 2 major topics within the domain of predictive analytics: “predicting quantities” and “predicting events”. Within the “quantities” part we will focus on linear models, variable selection and regularizations, as well as on time-series analyses. Within the “events” part we will focus on generalized linear models (logistic regression) and get an introduction to supervised machine learning (CART, random forest, boosting, and neural networks).
Technology in banking will cover the financial system and banking topics with a focus in technology induced changes. Open-banking that allows for the free flow of financial data in a manner that is controlled by the user, and not the financial institution will change how banks seek deposits, manage investments, and make loans. The impact of machine learning algorithm on lending and credit-scoring will be explored.
Distributed ledger technologies, like Blockchain and Ethereum, have changed how people view and transfer funds and how economics of the future will function. Central-bank issued digital currencies, Bitcoin and other crypto-currencies and tokens are changing the way that firms acquire funding. The course will cover the technology and economics driving these changes including consensus mechanism, use case and other application. These and other topics will be explored in depth during this course. This course will include joint sessions with Payments Canada that will include guest lecturers, a joint case study, and insight into the future of payments and payments regulation world-wide. Iso-labs will provide a payments sand-box for students to use.
This course provides a comprehensive overview of the design, the manufacturing, and the pricing of plain-vanilla and complex derivative instruments, as well as structured financial products based on these derivatives. This is a practical, hands-on, ‘how to’, course that places heavy emphasis on the broad array of methods that are available to market makers and buy-side market participants for the pricing of derivative instruments and the hedging of unwanted risk exposures, i.e., closed-form solutions, numerical procedures, and Artificial Intelligence techniques, and their application in a real-life setting using real market data.
The course shows how to unwind unwanted risk exposures using Data Science techniques, as well as market-specific risk measures. The course encompasses both the traditional finance (TradFi) and the decentralized finance (DeFi) settings, and spans ‘linear’, ‘non-linear’, as well as hybrid instruments. The course provides an enterprise-wide view of risk and risk management for financial institutions and buy-side market participants, and shows how to implement state-of-the-art methodologies for the assessment of market risk and counterparty credit risk exposures associated with derivatives trading books, and the estimation of economic and capital requirements to cover these exposures. Finally, the course explores recent developments in the regulatory environment surrounding derivative markets.
This course explores the profound implications of AI on business and society. The ethical and policy issues linked with the application of AI in business are covered in-depth, including such issues as overcoming the job displacement due to AI by job creation, ensuring the public good as AI pervades the new economy, and balancing privacy and transparency in AI related endeavors.
This course covers machine learning (ML) and AI methods and their implementation in state-of-the-art tools and packages. Through lectures, case studies, and readings, students will gain a broad understanding of ML concepts, and will understand how to use ML as a way of thinking. Students will also develop a proficiency in building ML and AI applications — using modern tools and packages to solve real-world business problems. This course will include an introduction to machine learning, data mining, and statistical pattern recognition.
Technical topics will include: supervised learning e.g., decision trees, Naïve Bayes, support vector machines, neural networks; unsupervised learning e.g., clustering, dimensionality reduction, recommender systems; best practices in building machine learning applications e.g., bias/variance theory, model evaluation, feature engineering and selection, hybrid models; and the practical implementation of ML applications using modern tools and packages e.g., R, Python, and cloud-based services.
This course will be delivered throughout the program. Students will identify a financial innovation and technology problem or opportunity within their organization, or in the industry in general, and develop a solution. The solution can be in the form of a prototype, report, start-up, or other form of deliverable. The course will include workshops, mentorships (mostly board members), mini-courses, and numerous presentations, and mile-stones throughout the year. Teams will be encouraged to collect real data to develop their solutions. Students will present their solution to a panel of industry experts during a final presentation day.
Experiential Learning
Experiential learning, or “learning by doing” is one of the most effective ways to learn. Students in Smith’s Master of Financial Innovation & Technology program not only master the theoretical concepts, but learn how to apply these concepts to real-life opportunities.
FinTech Speaker Series Events
- Blockchain and Crypto Currencies
- Digitalization of Finance
- Open Banking and Decentralized Finance
- Corporate Financial Innovation
- FinTech Venturing
- AI, ML, & Disruptive Technologies
Competitions
Students can apply their learnings to compete for cash awards in our:
- FinTech Hackathon
- FinTech Pitch Competition
Student-run Organizations
Students may have the opportunity to take leadership roles in:
- MFIT Student Executive
- Various Smith- and Queen’s-wide clubs
- QUAAF
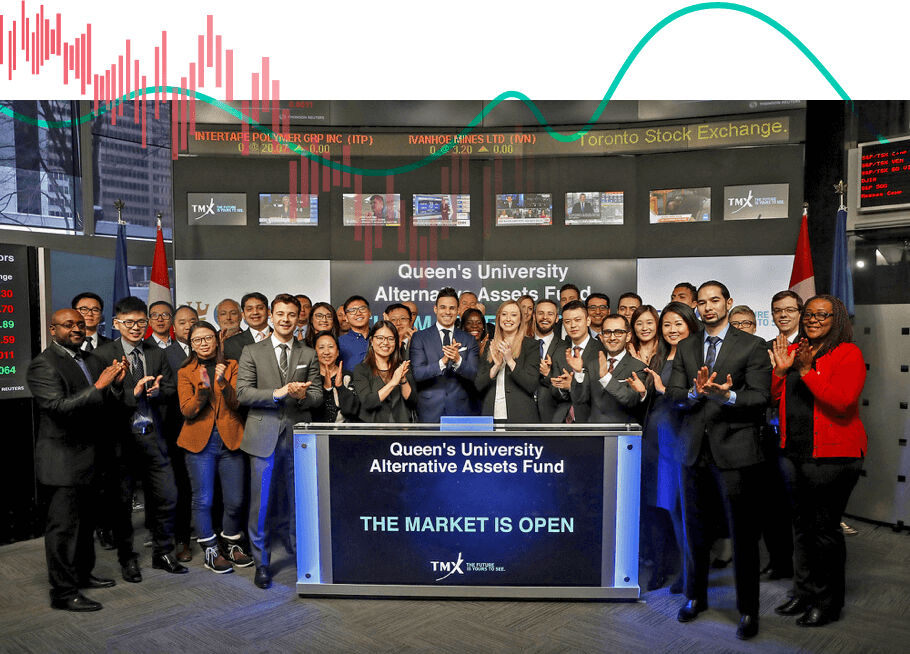
Queen's University Alternative Assets Fund
Students in the Master of Financial Innovation & Technology program have the opportunity to participate in the Queen’s University Alternative Assets Fund (QUAAF). It is the only student run hedge fund in North America and is comprised of an Executive Committee and teams of Analysts. They are supported by an Advisory Committee of industry professionals. This fund has been seeded with contributions from alumni and friends of Queen’s, and all proceeds contribute to the maintenance and expansion of the fund.
Learn more about QUAAFLive Data Sets & Technical Training
The program will also include access to finance specific data provided by industry. The data will allow students to work on current and relevant financial issues using state-of-the-art data science techniques.
Meet the Experts
Courses are taught by experts and range from importing data and data visualization to machine learning, deep learning and more.
View Faculty & Instructors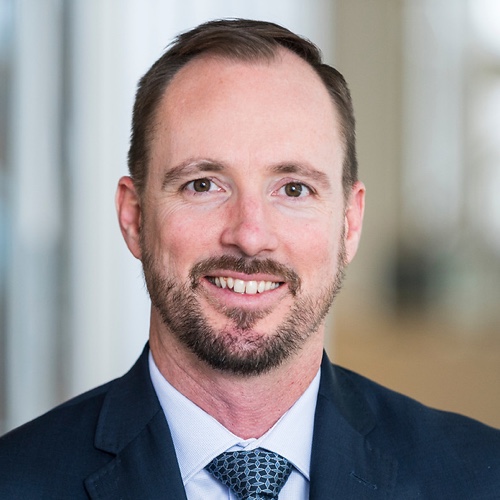
“The program enabled me to work in a role more related to strategy for digital product teams. I am now comfortable working more closely with specialized technology teams and distilling industry trends for conversations with executives. The end result for me is a foundation to more succinctly understand the ongoing evolution of technology’s impact on financial services - something I expect to accompany me in my quest to remain additive to stakeholders through the course of my career.”
Duncan C. Campbell, MFIT'22
Director of Business Development
Fincentric by S&P Global