How Technology Can Power Group Innovation
Discover how tech tools can help us find breakthrough ideas
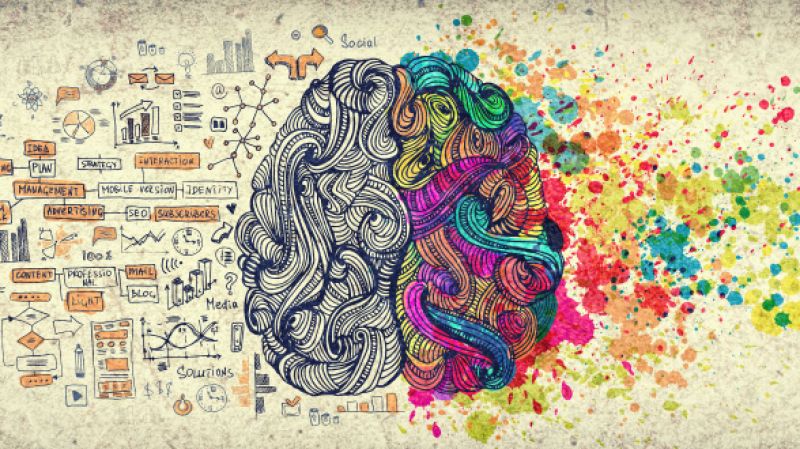
How hard can it be for a corporate team to dream up and decide on a couple of truly novel and feasible ideas? Assemble some smart people, set a clear mandate, give them a whiteboard and a few bottles of Yellow Tail, and let the brains start storming.
Apparently, not so simple. Innovating in a group setting requires team members who are comfortable with divergent thinking—their own and their colleagues’. They need the skill to explain the significance of their ideas and be willing to hear other options. When selecting a winning idea, it’s easy to see power dynamics emerge or information overload set in.
“If you revert to what you all know, you end up with a very mediocre idea because you’re not really leveraging the deep expertise of various team members,” says Tracy Jenkin, an associate professor at Smith School of Business, whose research focuses on digital technology, artificial intelligence and analytics.
Going with the familiar can result in “shallow, surface-level ideas that are understood by all members of the group but are not particularly novel. Or the novel ideas that are generated might only be understood by one or two members,” Jenkin says.
Clearly, in seeking the truly novel, innovation teams can be undone by human biases and group dynamics. That’s why Jenkin is bullish on the potential of technology tools to help humans overcome these roadblocks as they search for breakthrough ideas.
Looking for an innovation trigger
Among the most popular tech tools for group divergent thinking is electronic brainstorming, which allows team members to share and discuss ideas anonymously. They don’t have to wait for colleagues to stop talking before submitting their ideas or be inhibited by concerns for how they’ll be viewed by others.
But Jenkin identified gaps in what these tools offer. Electronic brainstorming and similar supports for divergent thinking are not designed to help brainstormers tap into inspiration from external sources—beyond fellow team members—that can be recombined into something that has never been considered before. And there are even fewer tools to support the convergent thinking essential for winnowing out the ideas. This tough love is often done by a panel of outside experts or enabled by decision support systems based on democratic voting. Democracy, however, is a flawed way to judge the potential value of a novel idea.
Over the years, Jenkin has worked with Queen’s University colleagues Yolande Chan (now at McGill University) and David Skillicorn to develop text- and data-analytics-based tools for group innovation. The researchers tested two in a field study with a team of six people, working in a variety of roles and departments, at a Canadian subsidiary of a Fortune 500 high-tech company. Team members were asked to develop and agree on two ideas for how to generate business value from emerging technologies.
Search engine for novelty
To help come up with ideas, the researchers had the team use a web-based search tool called Athens that was developed in earlier studies. Athens is like Google in that it’s a search engine delivering results from selected queries. Unlike Google, though, the results are only faintly related to the original query. A search for “virtual reality”, for example, may return “injury rehabilitation”, as it possibly would if you were following bread crumbs in a dream state.
As Jenkin explains it, Athens is based on the idea that people discover novel ideas when they’re exposed to a broad range of information indirectly related to their topic and—locked in the proverbial confusion room—are then forced to make sense of the results.
In the field test, each team member used Athens to trigger initial ideas. Jenkin then put the team through two rounds of discussion and anonymous rating of all the options, based on the “radicalness” and potential benefit of each idea. Finally, in a democratic vote, the group selected two to present to management.
For team members who were, by nature, comfortable with free-flow thinking and zigzag pathways to ideas, Athens was like a superpower. Those more literal-minded struggled to make sense of the creative clues Athens provided. Generally, though, team members weren’t so stuck on the ideas that they themselves had come up with. “There was less ego,” says Jenkin. “Rather than defending ideas, people appeared more inclined to consider and filter ideas during the convergent phase.”
Borrowing from Amazon and Netflix
Jenkin wanted to see if a collaborative filtering (CF) tool would come up with similar results if fed the same ratings that the group members anonymously gave for each idea. Collaborative filtering is the basis of recommender systems used by Amazon, Netflix and a slew of other online platforms. It uses data analytics to make predictions about an individual user’s interests based on the preferences of many users.
The CF tool developed by the research team analyzes the ratings that team members give prospective ideas and then weights each person’s judgment. It discounts the opinions of apparent “weak” assessors and boosts the opinions of “strong” assessors. This weighting is based on the degree to which an individual’s opinions line up with the opinions of other individuals who have demonstrated strong judgment.
“For example, the conventionally best movies are known as ‘blockbusters’ that are universally popular—most people like them, but often only weakly,” Jenkin says. “The worst movies are also universally regarded as bad. However, it is often more interesting to look at movies that are liked by a relatively smaller number of discerning movie critics. These movies do not appeal to everyone but are regarded as excellent by a small number of individuals with high-quality movie-rating skills.”
In their study, the researchers compared what the innovation team came up with on their own with what the CF tool identified as the top ideas. The results of the two approaches partly overlapped but there was one idea the CF tool determined as a winner that did not make the final cut for the group.
Identified in the study as “idea 13”, the innovation was an industry-specific application and consulting opportunity, quite unlike the broader product development ideas chosen by the team. Jenkin says team members may have overlooked idea 13 because it didn’t fit into the existing cluster of ideas. Unconscious bias or information overload may also have been a factor.
“When we shared these findings with the company sponsor of the project,” says Jenkin, “she said that without the insights from the CF tool, idea 13 might never have come to light as something worth pursuing. It would have left a valuable idea on the table.”
The human role
As this field study shows, a divergent thinking tool such as Athens requires training for individuals less open to exploration. Many of us seek a straight line toward a novel idea, Jenkin says, and close ourselves off to inspiration that arrives out of the blue. A collaborative filtering tool requires proper framing as well. It’s not about letting algorithms dictate which ideas will win out; it’s more about overcoming the challenges of group innovation by using collaborative filtering alongside group discussion.
“We’re all humans with limitations,” says Jenkin. “We’re all different in how we rate things. Can we get algorithms in the loop as a second check to ask, Have we missed anything? Have we fallen into groupthink or bias?”