He Analyzes, He Scores
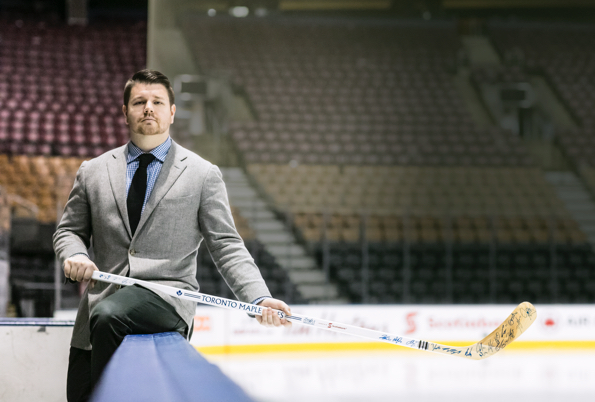
In Moneyball, the 2003 book by Michael Lewis and 2011 film starring Brad Pitt, baseball manager Billy Beane turned the cash-poor Oakland A’s into big-league contenders with help from analytics. By studying player data, Beane was able to spot good but inexpensive players overlooked by old-fashioned scouting.
But analytics isn’t just changing sports on the field. It’s impacting the business side, too. Case in point: Maple Leaf Sports & Entertainment Ltd., owner of the Toronto Maple Leafs, Raptors, soccer’s Toronto FC, as well as several other sports franchises, entertainment properties and restaurants. MLSE has a team of 17 people working in analytics and research for its businesses, led by Smith alum Sean O’Brien.
Sean, 33, grew up in Toronto; his dad an electrician and his mom an office manager. A big sports enthusiast, Sean played Junior C hockey until he was 19, then headed to Queen’s to study economics, taking, as he puts it, “a whole lot of math and statistics courses” along the way.
Upon graduation, Sean was hired by MLSE’s ticketing department. Eventually he started doing analysis and research and, in 2016 (a few years after gaining his Master of Management Analytics degree at Smith), was named MLSE’s Director of Analytics and Research. Big data, machine learning and complex coding all factor into his team’s work. But Sean says his job is really to “turn data into information, information into analysis, analysis into insights, and insights into strategy.”
Smith Magazine spoke with Sean about his career, analytics and the surprising role that creativity plays in data research.
How did you start working for Maple Leaf Sports & Entertainment?
My goal, after I got my undergrad in 2009, was to work in finance. But the financial crisis hit and there weren’t a lot of finance jobs. Every day I’d go onto the Queen’s career service board to see what was out there. One day this financial reporting position with MLSE came up. I thought, that’s really cool, I could do that. I printed a hard copy of my resumé right away and drove it down to reception at MLSE in Toronto. So the day the job was posted, they already had a hard copy of my application in their hands.
So what did your first job entail, and how did you go from there into analytics?
I started in ticketing. It wasn’t a high-level position, but it allowed me to understand the ticketing business and the underlying databases involved. It was also a very manual job, so I figured a way to automate it and, from there, I began doing trend and price analysis in terms of understanding season ticket holders, mini-pack holders, single-game buyers and so on. Our analysis work on the ticketing side became the basis for MLSE’s investment in an analytics team to look at retail, marketing and other areas of the business. We started with four people, and now we’ve grown to 17.
How did you come to get your Master of Management Analytics degree?
I’d always planned to get my MBA. I figured I’d go away to the U.S. and do it. But then I learned about the MMA program at Queen’s. I called and spoke with [Smith analytics Professor] Yuri Levin. He asked me what I did, and I told him I do dynamic pricing for the Toronto Raptors. I asked him what he does, and he said he studies dynamic pricing. We really hit it off. The program was a great experience and ended up being a catalyst for what I’m doing now.
What areas of MLSE’s business do you concentrate on?
We work with every single business unit here. We work with the marketing department to decide which marketing channels are working and which aren’t. On the sponsorship side we do a ton of research to validate and measure the effectiveness of sponsor agreements. We also look at how to optimize ecommerce and understand the customer journey and fan experience. We even examine weather. Weather provides a lot of insight into human behaviour, like whether people decide to go out and see a game.
What other types of analysis do you do?
One area we’re focused heavily on is content personalization on our website, apps and social. So on our app, based on the preferences a person has chosen and the way they interact with the app, we use machine learning and deep learning techniques to analyze user behaviour to provide content that’s most relevant to them.
If you’re a basketball junkie, and you’ve shown you like to get the in-depth Raptors interviews, we’ll prioritize that content for you. For us, we’re not competing against the Cleveland Cavaliers or Pittsburgh Penguins. We’re competing for share of personal disposable time. If we offer you a more relevant app, you’re more likely to use it.
What about the fan experience at games? What kind of impact does your work have there?
I’ll give you an example: A while ago we wanted to minimize wait times in the food and beverage lines at our sports venues. We looked at the menus, which items were popular and which weren’t. We found that, as we cut away poor performing items, it improved the customer experience.
We also simplified the menu board with pictures for our most popular items, so people understood, for instance, what the burger they were ordering looked like, whether it would come with one patty or two. If we can help people figure out their menu options faster, they’ll have an easier time ordering, the line will move faster, we can prepare food faster. Then the fans can get back to the action quicker.
Is everyone on your team an analytics expert or are there other professions involved?
Our team has incredibly diverse backgrounds. We have someone with a PhD in astrophysics, a psychologist, a social scientist, someone with a master in computer science working in artificial intelligence. We focus heavily on diversity of thought and finding people who are bright but don’t necessarily think the same way, because creativity is important in what we do as well.
“Creativity” isn’t a word that comes to mind when I think of analytics. Why is that an important skill in your business?
It’s easy to create this dichotomy of math versus art, creative versus quantitative; someone who understands math can’t possibly be creative. And that’s just not true. We ask a lot of questions on our team and there’s a lot of discovery that goes on in what we do. Sometimes you have to be creative and entrepreneurial in how you arrive at decisions. A significant amount of our time is dedicated to finding solutions to things that perhaps we didn’t even realize were problems.
You’ve spoken about communications as a necessary skill for people working in analytics, too. Why is that important?
Most people who work in analytics are extremely passionate about the math, the coding. We love to talk about that stuff. We’re like car enthusiasts who get excited talking about carburetors and camshafts. But most car buyers just want to know the car is an Audi, it’s black, it has eight cylinders. So, in our case, when we communicate about what our team is doing internally to executives and others, we really try to bridge the gap between analytics and strategy. We don’t focus on how complicated our machine learning algorithm is. We focus on the drivers of the business and make it actionable — this is how many staff you should have at the gate, these are the optimal marketing dollars to spend. Our job is to translate data into insights. Being able to communicate what the numbers mean is important.
Any advice for MMA students graduating in the future?
Yes. Start a company. My wife is an entrepreneur and watching her build a company is really exciting. It’s never been cheaper or more effective to start your own business. So the best advice I have is to start a company in an area you’re passionate about. This technology revolution we are going through is completely changing the possibilities for businesses of the future.